Building and Outsourcing High-Performance Data Science Teams: A Comprehensive Guide
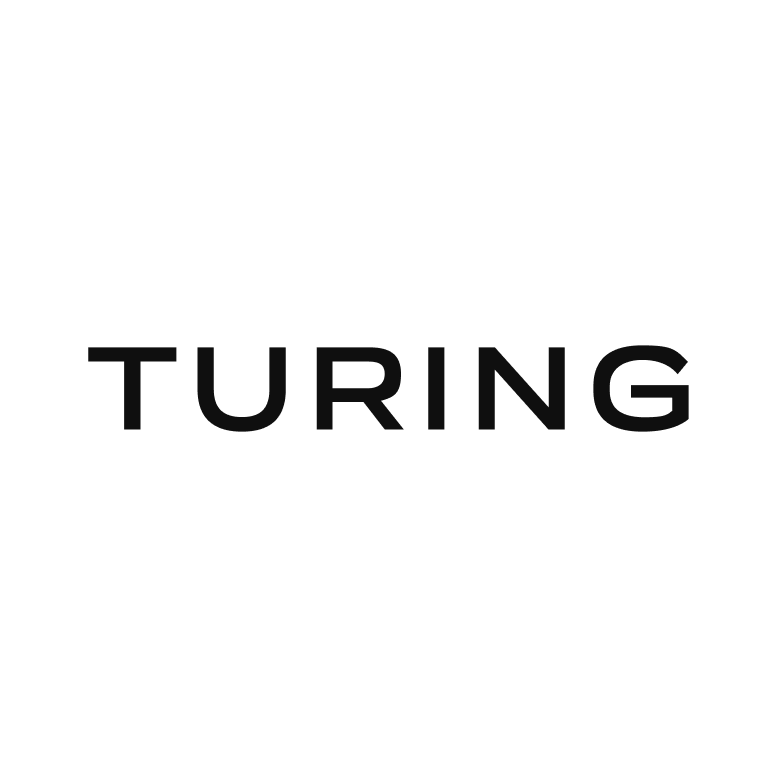
Turing Staff
•5 min read
- Hiring vetted talent
Data is extremely critical to the success of a business. In fact, it empowers companies to better their key strategic initiatives. A survey commissioned by Deloitte Analytics interviewed senior executives at 35 companies in the United States, Canada, China, and the United Kingdom, where 49% of respondents asserted better decision-making capabilities are the key advantage of data and analytics.
This is where the role of data science becomes critical. It plays a fundamental role in harnessing the power of machine learning, AI, predictive modeling, etc. Through data science, businesses can extract valuable insights for better decision-making, running machine learning models, and delivering cutting-edge applications. However, building a proficient data science team requires more than just hiring top talent; it demands attention to team culture, organizational structure, and a blend of diverse skills and roles.
This comprehensive article explores the dual approach of building high-performance data science teams in-house and augmenting capabilities through data science outsourcing.
So without further ado, let’s get started!
Building a data science team
Creating a high-performance data science team is a strategic endeavor that involves multiple facets, from deciding on the team composition to ensuring the team has access to the necessary tools and technologies.
Team structure and essential roles
A data science team's success largely depends on its composition, which includes a range of critical roles each contributing unique skills:
- Data scientists and analysts are at the heart of the team, tasked with data collection, preprocessing, and analysis to solve complex issues and identify trends.
- Machine learning engineers and AI engineers focus on developing, deploying, and maintaining models and algorithms essential for AI and ML initiatives.
- Data engineers specialize in constructing and maintaining the architecture of data pipelines to ensure the smooth and efficient flow of data.
- Data visualization specialists are experts in translating complex data findings into clear, actionable visual formats to inform decision-making.
- Domain experts provide invaluable insights into the specific industry to enhance the relevance and accuracy of data analyses.
- Project managers are crucial for overseeing the progression of data projects to ensure they are aligned with business objectives and completed within the set timelines.
Best practices for effective team building
Building a data science team is easier said than done. Even after implementing the best hiring strategies, using cutting-edge recruitment platforms, and following industry trends, companies often struggle to build a consistently successful team.
Here are four best practices companies can follow when building a data science team:
- Clear objectives: Starting with a clear vision and strategic objectives is critical for guiding the team’s efforts and aligning them with the business goals.
- Diversification of skill sets: A team composed of members with varied expertise can tackle multifaceted challenges more effectively, offering comprehensive solutions.
- Robust data management: Ensuring data is managed securely and efficiently is fundamental and requires strong governance practices to support the team's work.
- Collaborative culture: Promoting open communication and collaboration within the team and with other departments facilitates the seamless exchange of ideas and insights.
Leveraging outsourcing for enhanced data science capabilities
Given the complex nature of data science projects and the competitive landscape for hiring skilled professionals, outsourcing has emerged as a practical solution for many organizations.
The strategic advantages of data science outsourcing
Outsourcing can overcome challenges such as talent shortages and the need for specialized knowledge. It provides scalability, flexibility, and access to global expertise that allows companies to leverage the latest technologies and methodologies without the overhead associated with expanding in-house capabilities.
Navigating the potential drawbacks
While outsourcing offers numerous benefits, there are potential pitfalls such as communication barriers, loss of direct control over projects, and concerns about data security. Careful selection of outsourcing partners, clear communication protocols, and stringent security measures can help mitigate these risks.
Integration strategies for outsourced and in-house teams
Successfully blending the strengths of both in-house and outsourced data science capabilities requires thoughtful strategy and management. This includes defining clear roles and responsibilities, establishing communication channels, and fostering a culture of collaboration and innovation across all team members, regardless of their location.
The dos and don'ts of outsourcing data science services
It is crucial to establish guidelines in order to successfully manage your outsourced service provider. Here are some do’s and don’ts that you must heed when outsourcing data science projects:
Do’s:
Do: Ask the right questions
Given the plethora of service providers, selecting the right one can be highly confusing. To minimize risks and potential costs, you must ask the right questions and make informed decisions. Here are a few questions that you can begin with:
- Whether or not they have a positive track record in the industry?
- Do they have skilled and experienced teams who can successfully lead your project?
- Do they have any portfolios and references?
- What tech stack they’re using?
- Does their company culture align with yours?
Do: Protect your data
Data is critical and can be damaging to the company if compromised. Implement best practices and guidelines for data breach prevention. For instance, execute regulatory compliance, involve legal backup, invest in advanced security solutions, lay down administrative measures, and conduct regular security audits.
Don'ts:
Don’t : Concentrate entirely on the cost
You must not automatically select the cheapest option. After all, quality work comes with a price. You must do your due diligence and look at all the aspects before you decide on partnering with a particular data science services provider.
Don’t: Block access to crucial information
You will have to proceed with caution when outsourcing data science. Find the right balance between protecting your data and providing access to essential information. Establish the right safeguards and form an exit strategy once you end the partnership.
Conclusion
Developing a data science capability, whether entirely in-house, outsourced, or through a hybrid approach, demands thoughtful planning and strategic execution. By understanding the critical roles and best practices in team building and the strategic benefits and considerations of outsourcing, companies can pave the way for enhanced innovation, efficiency, and competitive advantage in the data-driven digital era.
At Turing, we offer comprehensive and end-to-end data science services, including data collection and preprocessing, data pipeline, analysis & visualization, machine learning, and predictive analytics, among others. Having served over 1000 companies across 40+ industries, Turing acts not just as a leading tech service provider but as a strategic partner in your data transformation journey. With access to over 3 million professionals, Turing ensures your organization is future-ready, turning challenges into innovations.
Get started today!
Want to accelerate your business with AI?
Talk to one of our solutions architects and get a complimentary GenAI advisory session.
Get Started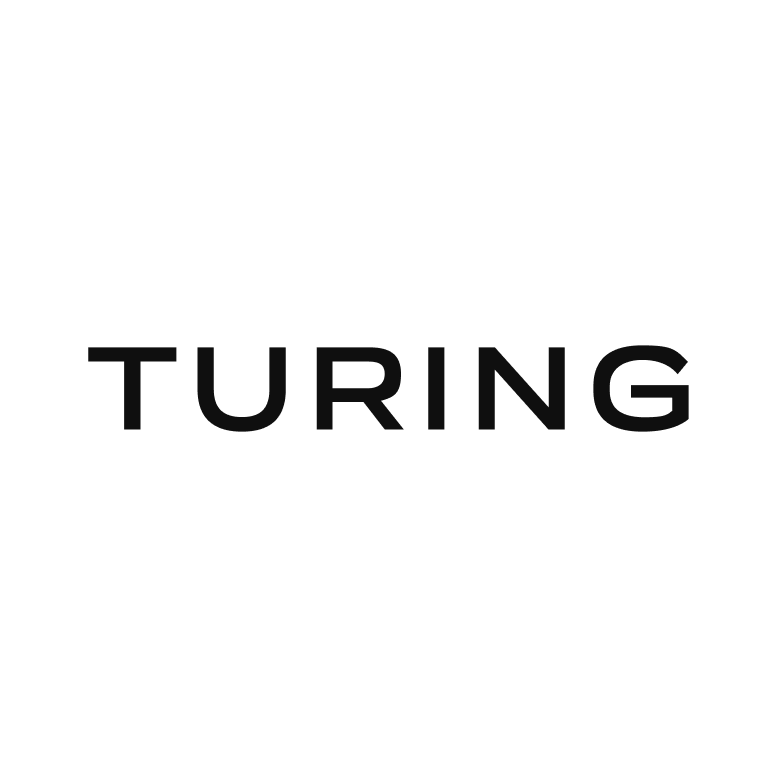
Author
Turing Staff