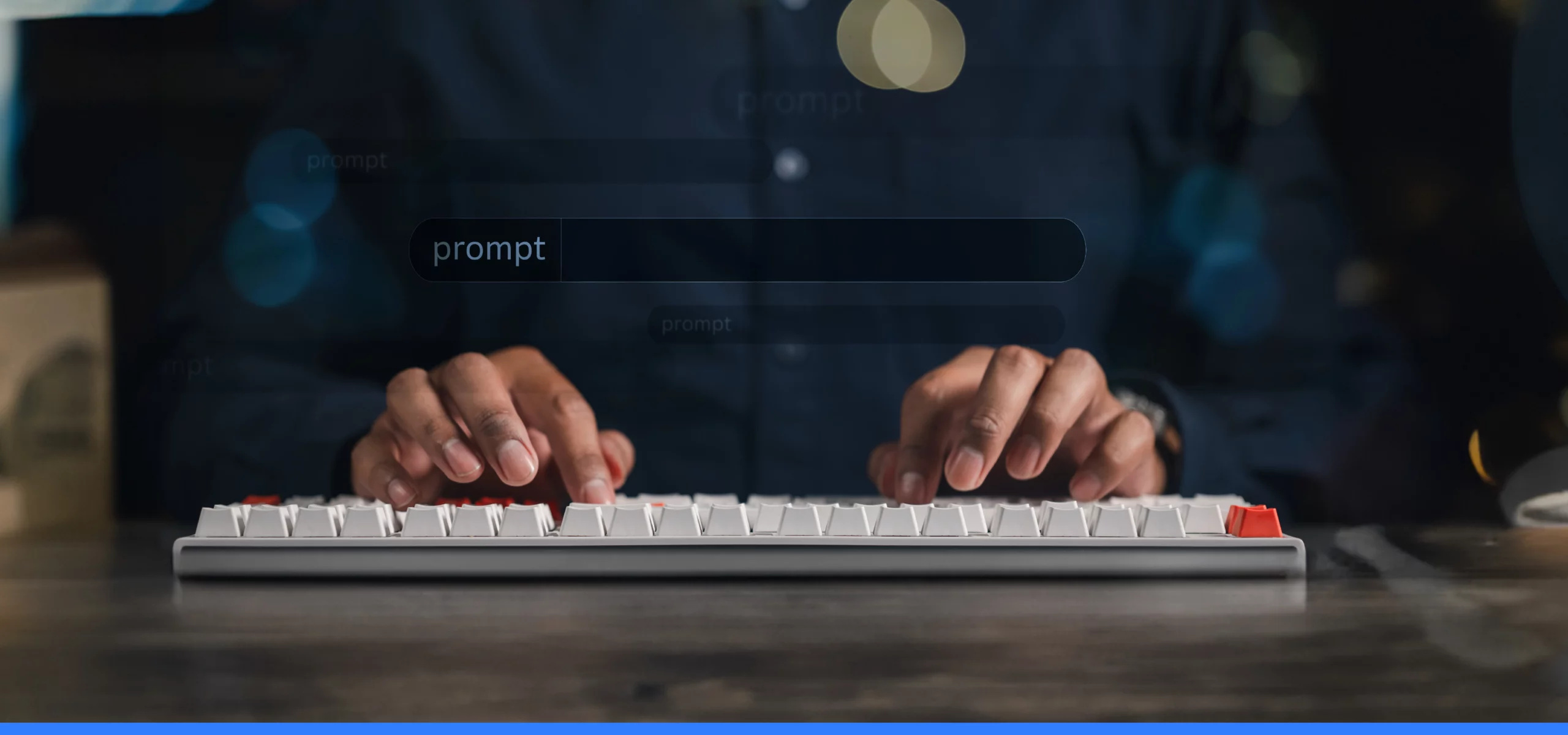
Mastering Prompt Engineering Skills: Bridging Human Intent and Machine Comprehension in AI
With the evolution of AI, sophisticated large language models (LLMs) have emerged that are capable of processing vast amounts of data and generating responses for users. Tools like ChatGPT and other generative AI (Gen AI) systems have revolutionized the way people work, study, and access information.
However, effectively utilizing these tools requires more than just their existence. AI lacks inherent abilities to understand user intent without clear instructions. It relies on prompt engineering—the art of crafting precise queries—to deliver meaningful results. As a result, there has been a surge in demand for prompt engineers who specialize in formulating the right questions to extract desirable insights from AI systems. These AI prompt engineers possess the unique skill of translating human intent into language that machines can comprehend.
Through this article, we’ll delve into the key prompt engineering skills that aspiring prompt engineers should cultivate to excel in this dynamic field.
Skills needed to become a prompt engineer
Programming proficiency
Although prompt engineering is not similar to programming, prompt engineers are required to have some coding knowledge. A proficiency in Python enables them to understand the details of the AI models wherein they can fine-tune algorithms and optimize performance.
In addition, having a robust understanding of APIs, operating systems, and command-line interfaces (CLIs) can significantly enhance one’s capabilities as a prompt engineer. The specific requirements may vary depending on the company and the AI platform being utilized.
Computer science fundamentals
A strong foundation in computer science is indispensable for prompt engineers. Having the knowledge of data structures, algorithms, and software development equips the engineers with tools to create, train, and deploy AI models.
Critical thinking
Critical thinking is a cornerstone skill for AI prompt engineers, enabling them to better evaluate AI outputs. By analyzing patterns and identifying inconsistencies within data, prompt engineers can refine AI models for enhanced performance.
Critical thinking fosters a problem-solving mindset that empowers engineers to overcome challenges and drive innovation in prompt engineering.
Effective verbal and written communication
Prompt engineers need to communicate to AI systems using carefully chosen words and phrases. Crafting detailed prompts can be complex, sometimes requiring hundreds or even thousands of words. Additionally, because prompt engineering involves different fields, good communication and teamwork are essential for success.
These skills allow prompt engineers to articulate technical concepts to non-technical stakeholders with clarity and precision. This in turns fosters collaboration and understanding between all teams and ensures that everyone involved understands the goals, limitations, and implications of AI solutions.
Proficiency in AI technology
Prompt engineers leverage language skills alongside a deep grasp of natural language processing (NLP), large language models (LLMs), machine learning, and a basic understanding of AI-generated content development. This knowledge is crucial, especially if the engineer will be engaged in coding or other responsibilities related to AI platform development.
Data analysis proficiency
Prompt engineers must comprehend the data utilized by an AI platform, including input data, prompt data, and AI-generated output. This necessitates a solid grasp of data analytics techniques and tools.
Conclusion
In the rapidly evolving landscape of AI, prompt engineers play a pivotal role in bridging the gap between human intent and machine comprehension. From programming proficiency to critical thinking and effective communication, prompt engineers must embody a blend of technical prowess and soft skills. They are not only tasked with crafting precise queries but also with refining AI models to ensure their efficiency and relevance.
Furthermore, proficiencies in AI technology and data analysis are indispensable for prompt engineers to navigate the complexities of AI platforms and extract meaningful insights from data. These prompt engineering skills empower them to optimize performance, mitigate biases, and drive innovation in the field of prompt engineering.
As demand for AI prompt engineers continues to surge, cultivating these essential skills will be crucial for aspiring professionals looking to excel in this dynamic and impactful domain.
Join a network of the world's best developers and get long-term remote software jobs with better compensation and career growth.