Top Generative AI Industry Applications: An In-Depth Look
•13 min read
- GenAI
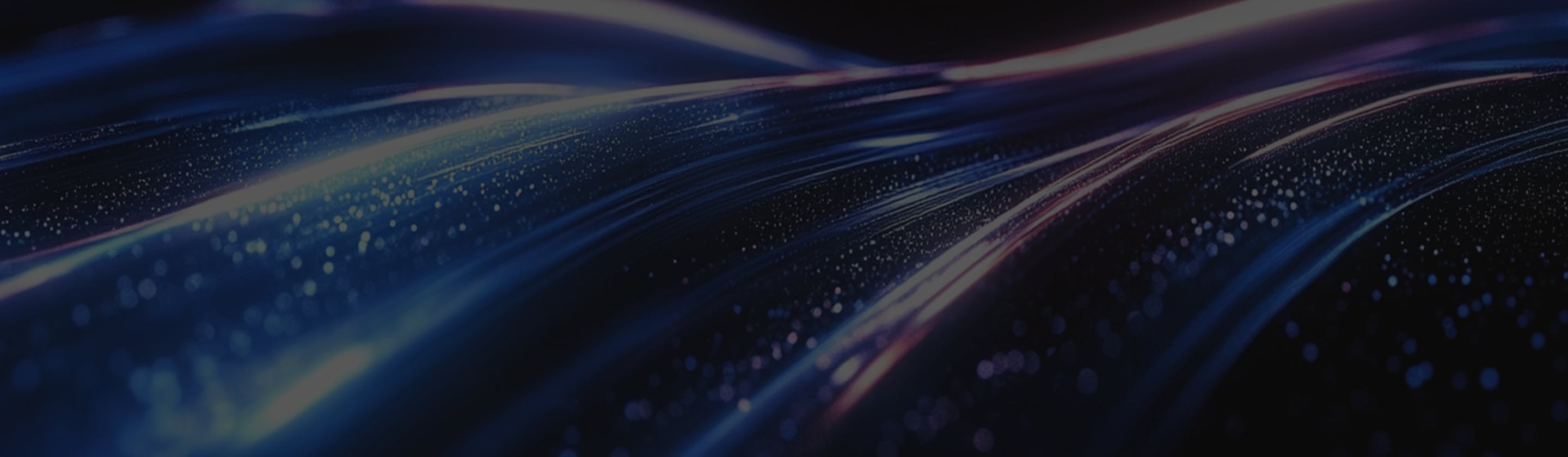
The overall AI landscape took a significant turn with the arrival of powerful generative AI models, resulting in the mainstream adoption of automation. Consequently, generative AI has captured the attention of numerous organizations, prompting questions about its transformative capabilities, and more importantly, real-world use cases.
So, what are the most important generative AI applications today? How does this new-age technology operate? In this blog, we aim to answer these critical questions and provide a comprehensive overview of the applications of generative AI, its benefits, the reasons behind its rapidly-growing popularity, and more.
Let’s get started!
Understanding generative AI
The advent of prominent generative AI tools like ChatGPT and Midjourney has prompted many to better understand what generative AI is. Moreover, generative AI applications and tools are empowering both organizations and individuals to automate tedious tasks, make better decisions, and streamline operations for maximum efficiency. Here’s a deeper look into generative AI, its benefits, models, known risks, and popular examples.
1. What is generative AI and how does it work?
Generative AI is a subset of AI that uses machine learning techniques like semi-supervised or unsupervised learning algorithms to create digital content like images, audio, videos, codes, or texts. This is done through training, where algorithms are supplied with large datasets of output/input examples to obtain patterns from the input that result in conclusions about the desired output.
Today, generative AI applications primarily involve generative AI models being trained to create content as responses to natural language requests. This doesn’t require any experience or knowledge of coding. In a nutshell, generative AI begins with prompts that could be texts, images, designs, audio, or any other input that the specific AI system can process. AI algorithms then return new content in response to the provided prompts.
2. Why is generative AI quickly gaining popularity?
The early versions of generative AI involved submitting data through APIs or other complicated processes. Developers often had to learn the ins and outs of specialized tools and write applications via Python or other languages.
However, late 2022 witnessed a surge in generative AI’s popularity, with the arrival of ChatGPT. One of the most prominent generative AI applications, OpenAI’s ChatGPT is a chatbot capable of highly human-like interactions. ChatGPT paved the way for the wide adoption of generative AI tools, where an increasing number of people and organizations started using these tools for various needs, from writing essays to transforming business operations.
Currently, Hootsuite reports 100 million+ Americans will use generative AI by 2024, and the number is predicted to reach 116.9 million by 2025. This raging popularity of generative AI is primarily due to the vast benefits it offers. Generative AI applications are designed to enhance customer experiences, expedite product development, boost employee productivity, deploy customized and innovative content, and more.
3. The known risks of generative AI
As beneficial as generative AI is, it also comes with major limitations due to the technology being in its nascent years. Here are some of the most known risks of implementing generative AI:
- Lack of transparency - Generative AI models can be unpredictable in their responses, and those using such models may not always understand how they or applications based on these models operate. This lack of transparency makes generative AI applications a bit troublesome to work with.
- Biased responses - When using applications of generative AI or generative AI models, it’s important to have controls or policies in place to detect biased responses to ensure their appropriate usage. For instance, if a business is using a generative AI platform, it must have controls to detect biased outputs to let employees deal with them in a manner aligned with the business policies.
- Inaccuracy - Generative AI models, still in the early stage of development, often produce fabricated and inaccurate responses. Hence, when implementing them for generative AI use cases, one must assess responses for appropriateness, usefulness, and accuracy before relying on them or distributing the information.
- Privacy concerns - Generative AI models may also inadvertently memorize and recreate content around private or sensitive information from their training data. This can compromise individual security and privacy and is a major concern for enterprises implementing generative AI applications.
- Intellectual property (IP) issues - Generative AI models can also be trained on proprietary or copyrighted data. If so, there could be ethical and legal concerns regarding the ownership and usage of the generated content.
4. The most popular examples of generative AI
While generative AI has its drawbacks, its benefits and future potential far outweigh them. Some of the recent generative AI applications have proven how this new-age technology can help with innovation and creativity, indicating usability for both businesses and individuals. Some of the popular examples of generative AI are the following applications:
- ChatGPT- One of the major drivers behind the worldwide popularity of generative AI applications, ChatGPT is a chatbot built by the Microsoft-backed AI research organization, OpenAI. This AI-powered chatbot took the world by storm, thanks to its human-like responses, starting with OpenAI’s GPT 3.5 implementation. Now, GPT-4 has been released, offering a more seamless interface with better AI capabilities for more accurate responses. ChatGPT’s massive popularity earned it Microsoft’s investment - a significant one - and the tech giant even incorporated GPT into its Bing browser.
- DALL.E - One of the first generative AI tools to be widely adopted, DALL.E is another OpenAI creation, built through GPT implementation. DALL.E is a multimodal AI application, trained on a vast amount of images and their text descriptions, that can identify connections across various media like text, audio, and vision. In this case, DALL.E connects words to visual elements, meaning it can generate images from user prompts.
- Bard- Google has also been an early leader in facilitating transformer AI models for various types of content, including processing language. However, Google never released a public interface for AI models, until Microsoft used GPT in Bing, which prompted Google to also launch its own chatbot, Google Bard. Bard’s initial run was devastating due to overall AI platforms’ erratic behavior and inaccurate responses. However, Google has since released a new Bard version, built on PaLM 2 (Google’s most advanced LLM) which allows the chatbot to have higher efficiency and offer more visual responses to prompts.
- Midjourney- Midjourney is another prominent example of generative AI that generates images from natural language prompts. While Midjourney is one of several machine learning-based image generators to have emerged recently, it has quickly become one of the most preferred generative AI applications alongside DALL.E. One of the biggest reasons behind this is Midjourney’s ability to generate high-quality images from simple text prompts, allowing lesser-experienced users to easily access excellent images for digital use.
Key generative AI applications
While chatbots like ChatGPT and Google Bard have quickly risen in popularity, there are other generative AI use cases that are becoming prominent. Here are some of the most significant applications of generative AI that are being widely implemented today.
1. Image generation and manipulation
One of the most common use cases of generative AI is image generation, which is typically text-to-image conversion. Here, users can enter a textual prompt describing what type of image they want, and the AI tool will process the input to generate realistic images. When using such generative AI applications, users can specify subjects, styles, settings, locations, or objects to generate the exact images as per their requirements.
Apart from text-to-image AI applications that generate realistic images or 3D models, there are tools that facilitate image enhancement and manipulation, letting users modify existing images. Some of the major functions such tools can perform are:
- Semantic image-to-image translation - Creating realistic versions of an image based on semantic photos or sketches.
- Image completion - Generating missing portions of an image, such as filling in backgrounds with objects, people, or other elements. AI tools with this capability can also fix torn photographs or fill in missing pixels.
- Image super-resolution - Enhancing the resolution of images without any pixel-tear or other aspects that can cause loss of detail.
- Image manipulation - Altering or modifying existing images. For example, users can transform an external element of an image, like its color, lighting, form, or style, while maintaining its original elements.
2. Software and coding
Generative AI applications have already begun transforming the software development and coding landscape through innovative solutions that streamline coding. Hence, software and coding have quickly become one of the most prominent use cases of generative AI, as its applications hold the potential to improve code quality, enhance productivity, and even spark new software innovation avenues.
Here’s how applications of generative AI are impacting software and coding:
- Code generation - One of generative AI’s most prominent applications in software development is code generation. This involves training AI models on vast repositories of existing code, allowing them to generate code functions, snippets, or even entire programs based on prompted requirements. Code generation through generative AI applications proves invaluable in accelerating software development by automating repetitive coding tasks, and letting developers focus on problem-solving and higher-level design.
- Code completion - Generative AI can also boost coding efficiency by offering intelligent code completion and suggestions. IDEs (integrated development environments) can leverage generative AI models to predict future code lines that developers may write based on the context, expediting the coding process and reducing the possibility of error.
- Natural language interfaces for coding - Generative AI also enables natural language interfaces to code, allowing a developer to interact with software systems through human language instead of programming languages. Many organizations implement this through generative AI applications to bridge the gap between domain experts and developers. In turn, this helps to save resources on hiring experts to tackle software systems, by simply letting developers do it.
- Automated testing - Generative AI-powered tools can automate test case and scenario generation which is generally quite time-consuming during a software development lifecycle. Such tools analyze code and its probable execution paths to generate comprehensive test suits, thus enhancing code coverage and allowing developers to identify potential bottlenecks early on.
3. Video creation
Generative AI applications also simplify video production through highly flexible and efficient features that generate high-quality video content. Using generative AI models, applications can automate tedious tasks like video compositions, and animations, adding special effects, editing video snippets, etc. Like image generation, generative AI tools for video production can create videos from scratch, which can be used for enhancing video resolution, video manipulation, and completion.
Video generation AI tools can also perform:
- Video style transfers - AI video tools with this feature can generate new videos that follow the same style as another reference image or video.
- Video predictions - AI tools with this capability can predict the next frames in a video, using generative AI models. Such tools understand a video’s spatial and temporal elements, producing future sequences based on that data.
Besides video generation, generative AI applications are also helpful for 3D shape generation, where they’re used to build 3D models and shapes through generative models. AI tools achieve this through techniques like autoregressive models, GANs (generative adversarial networks), and VAEs (variational autoencoders). This is especially helpful when creating highly-detailed shapes which may not be possible when manually creating a 3D image.
4. Audio generation
Another one of the widely implemented generative AI use cases is audio generation, where generative AI is used to expedite the process of creating audio. There are three major use cases under this category, which are:
- TTS generators - GAN-based TTS (text-to-speech) generators can generate realistic speech audio from a user’s textual prompts. TTS AI tools use extensive text and speech data to train machine learning models, which can then be tweaked to create high-quality audio from text. Moreover, such tools are often used in applications like speech-based interfaces, speech-enabled devices, and assistive technologies.
- Creating music - Making music has proven to be one of the most common generative AI applications today. Generative AI models can easily produce new music pieces and generate complete audio by learning the styles and patterns of the music a user inputs.
- STS conversions - STS (speech-to-speech) conversions involve generative AI creating new speech or voices via existing audio files, which is commonly implemented in audio-related AI applications. STS conversions have become massively popular in the gaming and filming industries, where professionals use AI tools with STS conversion capabilities to seamlessly create voiceovers.
5. Text generation and summarization
ChatGPT is one of the best examples of text-generative AI tools that creates and summarizes textual content from user prompts. Such tools utilize generative AI models and are trained on large data sets to generate updated and authentic content. Listed below are some of the most common use cases of generative AI applications used for text generation and summarization:
- Content creation - Generative AI models are extremely helpful in creating various types of written content, from blogs to marketing posts and social media copies. Plus, generative AI applications like ChatGPT also speed up the writing process by generating ideas, quotes, content outlines, etc.
- Language translation - AI developers can also fine-tune generative AI models for translation tasks, where the models can analyze texts in one language and provide accurate translations in another.
- Virtual assistants and chatbots - Generative AI powers virtual assistants and chatbots, letting them generate contextually relevant and natural responses in real-time user conversations. Creating chatbots like ChatGPT has become one of the biggest generative AI use cases. Such chatbots enhance user engagement and help businesses offer personalized assistance.
- Content aggregation - In addition to text creation, generative AI tools can automatically summarize bulk texts like research papers, news articles, blogs, and lengthy emails to help users get a concise overview of the content. This also includes document summarization that helps businesses streamline document-related tasks using generative AI models.
- Automatic report generation - In business intelligence and data analysis, generative AI can help summarize complex datasets and generate detailed reports. This simplifies decision-making and allows concerned stakeholders to better understand trends, patterns, and insights.
6. Organizational collaboration
The latest advancements in generative AI applications have also led to businesses achieving better team collaborations. Personal productivity tools like word processing and email can now be augmented via automation to boost the accuracy and efficiency of users, i.e., organization members.
An excellent example of generative AI’s collaboration enhancement capabilities is Microsoft implementing GPT-3.5 in Teams Premium, which uses AI to enhance meeting recordings. It automatically divides a recording into sections, generates titles, and adds personalized markers for better reference.
Another notable example is the wildly popular startup, Jasper.ai. This generative AI-powered can be used to automate tedious writing tasks, as its powerful automation capabilities allow it to generate complete texts for various purposes, from job descriptions to marketing copies, and more.
7. Chatbot performance improvement
While chatbots are one of the most prominent generative AI applications, the technology also contributes to enhancing chatbot performance and abilities. In turn, this helps to facilitate more engaging and effective interactions between chatbots and users, which is primarily possible through generative models and NLP (natural language processing).
Here’s how generative AI is currently implemented for chatbot performance improvement:
- NLU enhancement - Generative AI models help enhance a chatbot’s natural language understanding (NLU). Training AI models on vast amounts of text data enables them to learn intricate language patterns, context, and nuances. This allows chatbots to better understand user inputs, accurately extract intent, and determine entities.
- Human-like response generation - One of the biggest benefits of generative AI implementation is allowing chatbots to generate human-like text. This has also become one of the most common generative AI applications, where it’s used to train a chatbot on a diverse range of conversations to learn how a human expresses themself. In turn, this helps the chatbot generate natural, conversational, and tailored responses.
- Handling open-ended prompts - Traditional rule-based chatbots often struggle with unfamiliar topics or open-ended user queries. Generative AI empowers chatbots to better handle such user inputs, even those they aren’t specifically programmed for. This elevated flexibility is achieved through training AI models on vast conversational data, enabling a chatbot to generate plausible responses to a wider range of queries.
- User profiling - One of the most transformative generative AI applications has been implementing this technology to facilitate chatbots creating user profiles. Using generative AI, chatbots can analyze past conversations to understand user preferences and establish a user profile based on them. This helps chatbots to tailor responses and recommendations to users, offering a highly personalized experience and better user engagement.
8. Enterprise search
Lastly, one of the most recent generative AI use cases has been the enterprise implementation of this technology for streamlined search. Using generative AI, organizations can access information faster, as such AI models can be trained to securely read through all organizational documentation, like contracts, research reports, business trend analysis, and so on. Moreover, developers can train generative AI models to automatically highlight the important sections of a document and allow enterprise members to quickly access the information they need.
To sum up
Generative AI has shifted far away from being a mere advanced tech concept. Today, developers and organizations are actively implementing this technology to create generative AI applications that lead to business transformation, innovation, growth, and better scalability. From creating and completing videos to expediting coding and enhancing chatbots, the generative AI use cases are continuously expanding.
Turing’s generative AI development services are driven by in-depth expertise and continuous innovation that help us offer tailored solutions. Our team of AI experts leverages vast industry experience to ensure business transformation by harnessing the true potential of generative AI, aligned with customer needs.
1000+ fast-scaling startups and Fortune 500 companies have trusted Turing for their engineering needs and business transformation, and so can you. Talk to an expert today and get tailored solutions designed for rapid business transformation.
Want to accelerate your business with AI?
Talk to one of our solutions architects and get a complimentary GenAI advisory session.