Top 26 Machine Learning Applications You Must Know in 2024
•10 min read
- Languages, frameworks, tools, and trends
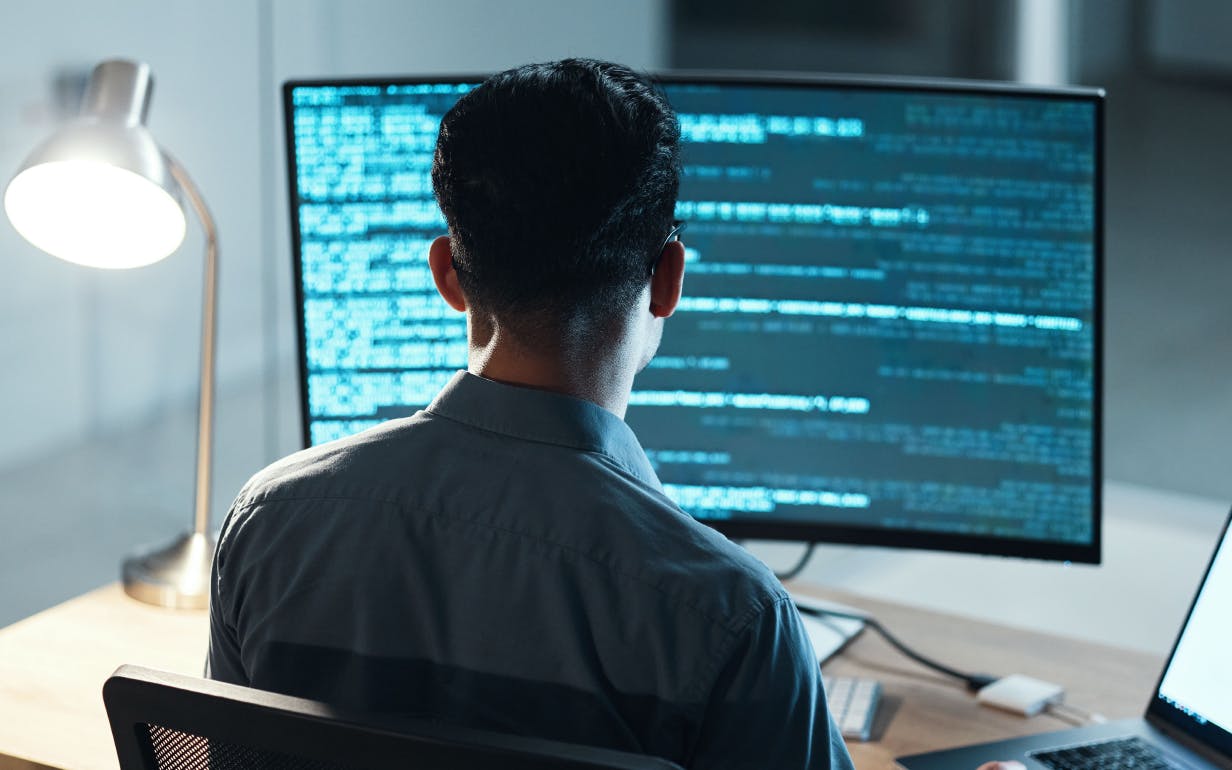
Machine learning is a modern breakthrough that has helped people better in a wide range of industrial and professional operations and their everyday lives. Machine learning applications are one of the most significant technological breakthroughs since microchips. But what are machine learning applications? How to use machine learning exactly? In this post, we will look at some real-world instances of machine learning applications and how it contributes to the development of better technology to improve the quality of our lives. Let’s get started!
Image recognition
Businesses are using algorithms designed to recognize patterns, such as machine learning, deep learning, and neural networks, to help them interpret data from images and photos. The use cases for this machine learning technology are numerous, ranging from Facebook's wish to tag uploaded photographs to security teams' desire to spot illegal activity in real-time to automated cars' requirement to observe the road. Retailers also use image classification and image recognition for a variety of purposes, such as the following:
- Enabling robots to check shelves for low-stock, out-of-stock, or misplaced items using computer vision and machine learning;
- Mitigating harmful situations by using visual analysis to spot shady behavior, such theft, and to find workplace safety violations, including illegal use of hazardous equipment.
Speech recognition
Speech recognition is converting spoken words into text. Also known as automated speech recognition or computer voice recognition. A software may recall what is said in an audio clip or file and convert it to text. Speech recognition is used in voice-user interfaces, voice search, and others. Device control is one of the machine learning examples of voice user interfaces. Speech recognition in automation provides driver safety by enabling voice-activated navigation systems.
Video surveillance
Video surveillance system powered by AI is used to detect crime before it occurs. AI-based video surveillance systems make it possible for users to sift through various actions or objects in all the footage considerably more quickly than a human operator could. As a result, the system may inform individuals, which can eventually aid in preventing failures.
Virtual PAs
Siri, Alexa, and Google Assistant are some well-known machine learning examples of virtual personal assistants. They assist you in getting almost every piece of information when asked for by the voice, as the name indicates. Simply activate them and inquire, "What is my schedule today?" “What are flights from Germany to London?” Or similar inquiries." Your assistant will hunt for information to answer your inquiries, recollect them, or issue an order to other resources (such as phone applications) to gather information. You may also train assistants to do specific duties like "Set an alarm the next morning," or "Remind me to go to the Visa Office every day."
Online fraud detection
Money fraud tracking is one example of machine learning applications to make cyberspace a secure environment. Online payment processors like Paypal, for example, use ML to prevent money laundering. It uses complex ML techniques to discern between legitimate and unlawful transactions between buyers and sellers.
Medical diagnostics
Machine learning applications can also be applied in disease diagnosis methodologies and tools. It is used to assess and aggregate clinical data in order to forecast disease progression, extract medical knowledge for research findings, therapeutic planning, and patient monitoring. These are some of the examples of successful applications of machine learning in the healthcare industry.
Statistical arbitrage
Using an automated trading approach called arbitrage, a lot of securities are managed in the financial sector. The tactic makes use of economic data and correlations to analyze a group of securities using a trading algorithm. Examples of statistical arbitrage in the real world are - Trading algorithms that examine the microstructure of a market, analyze a lot of data, and find prospects for real-time arbitrage. The arbitrage approach is optimized by machine learning technologies to improve outcomes.
Automating employee access control
Machine learning algorithms are being actively used by organizations to determine the level of access that employees would require in various locations based on their job profiles. This is one of the most intriguing machine learning applications.
Traffic forecasting
Traffic forecasting is one of the most effective machine learning applications. We use GPS navigation services almost every day. While we do that, our current locations and speeds are recorded on a traffic server. This information is then utilized to map the present traffic flow. This aids in prevention of accidents and analysis of traffic flow, the fundamental issue is that fewer GPS-equipped vehicles are on the road. In such cases, machine learning applications can assist in estimating areas where congestion occurs on a regular basis.
Information extraction
Another example of machine learning is information extraction (IE). Information extraction is the technique of automatically extracting the key information from text documents using popular machine learning methods like natural language processing. The technique extracts structured information from large and complex unstructured datasets. The extraction method generates structured data as a series of documents. Big data extraction is becoming a significant aspect. We are aware of the massive amount of data created, the majority of it is unstructured.
Agriculture
For high-quality produce, machine learning in agriculture provides accurate and effective farming with minimal labor. Additionally, machine learning offers priceless crop-related information and suggestions so that farmers can reduce losses.
Financial services
Machine learning applications have enormous promise in finance and banking. It is the driving reason for financial services' appeal. Machine learning may assist banks and financial organizations in making more informed judgments. As Machine learning applications can detect and respond to threats in real-time, it can assist financial services in detecting closure before it occurs. It can also track client spending habits. Market analysis may also be performed using machine learning applications.
Online customer support
Several websites now allow you to talk with customer service personnel while surfing the site. Not every website has a real executive available to address your inquiries. You're primarily conversing with a chatbot. These bots typically extract information from previous data and provide required information to website visitors. At present, chatbots are more likely to comprehend user inquiries and deliver better replies thanks to the machine learning algorithms.
Spam and malware filtering
Spam screening is used by several email clients. Machine learning applications are used to constantly update these spam filters. Some of the ML-powered spam filtering solutions include Multi-Layer Perceptron and C4.5 Decision Tree Classifier algorithm. Every day, over thousands of malware pieces are identified, and each piece of code is 90–98% similar to prior versions. The coding pattern is recognized by machine learning security tools. They easily identify and defend new viruses with a 2–10% variation.
Product recommendations
You purchased some goods online a few days ago, and now you're getting emails with buying ideas. If not, you may have noticed that the shopping website or app promotes things that match your preferences. Almost every online shopping platform uses machine learning applications that assist in improving your overall shopping experience. Product suggestions are based on your website/app activity, previous purchases, products liked or added to a basket, brand preferences, and so on.
Social media features
Machine learning algorithms and methodologies are used by social media platforms to generate some appealing and exceptional features. Facebook, for example, monitors and records your activity, conversations, likes, and comments, as well as the time you spend on various postings. Machine learning applications learn from your past experiences and suggest friends and pages for your profile.
Sentiment analysis
One of the most important machine learning uses is sentiment analysis. Sentiment analysis is a real-time machine learning program that determines the speaker's or writer's sentiment or opinion. For example, if someone writes a review or an email (or any other type of document), a sentiment analyzer will instantaneously determine the true thinking and tone of the content. Sentiment analysis techniques can be used to examine a review-based website, decision-making apps, and other similar applications.
Language Translation
Language translation is one of the most prominent machine learning apps. We are astounded by how websites can seamlessly translate from one language to another while also providing contextual meaning. The technology that powers the translation tool is known as 'machine translation.' It has allowed individuals to engage with others from all over the world. It has given vacationers and business companions the courage to walk into distant areas with the assurance that language would no longer be a barrier.
Search engine result refining
Machine learning application is used by Google and other search engines to enhance their search results for you. When you search, the algorithms on the backend monitor how you react to the results. If you open the top results and stay on the page for an extended period, the search engine concludes that the results presented were relevant to the query. Similarly, if you reach the second or third page of search results but do not open any of them, the search engine assumes that the results supplied did not meet your criteria. In this manner, the algorithms at the backend enhance the search results.
Intelligent gaming
Some people may recall the chess match between Gary Kasparov and IBM's Deep Blue, in which Deep Blue won. Or, more recently, in 2016, when Google DeepMind's AlphaGo defeated Go world champion Lee Sedol. Go, an ancient Chinese game, is considered far more difficult for computers to learn and master than chess. However, AlphaGo's AI was expressly educated to play Go by practicing against itself millions of times rather than just reviewing the moves of the world's greatest players.
Automated transportation
Today's flights employ FMS (Flight Management System), a mix of GPS, motion sensors, and computer systems, to track their position while in flight. When we apply the same approach to vehicles, the dynamics shift dramatically. There are other automobiles on the road, obstacles to avoid, and restrictions imposed by traffic laws. Nonetheless, self-driving cars are becoming a reality. According to a survey, more than 50 Google vehicles have traveled more than 1.3 million miles. These AI-powered cars can outperform their human counterparts. The introduction of Google Maps, which pulls position data from drivers' smartphones, has already fixed the navigation difficulties.
Public sector
Machine learning applications assist authorities in tracking and managing the massive volume of data collected by public surveillance systems. Law enforcement organizations use real-time data analysis to look for abnormalities and threats to hunt down criminals and missing children. As a result, it has become easier to detect cases of questionable online behavior.
Dynamic pricing
An old issue in economic theory is how to determine the appropriate price for a good or service. Numerous pricing tactics exist, and they vary depending on the goal being pursued. Everything is dynamically priced, whether it be a movie ticket, a plane ticket, or cab fare. Artificial intelligence has recently made it possible for pricing tools to track consumer trends and establish more affordable product prices.
Real-time weather forecasting
Big data allows us to access and store massive volumes of data, and AI could aid in identifying trends and using the data to create solutions to previously unsolvable problems. IBM's Green Horizon Project analyzes environmental data from various sensors and sources to generate accurate, real-time weather and pollution forecasts. Unique environmental friendly technology, such as self-adjusting smart thermostats and distributed energy grids, are routinely introduced to the market.
Better elder care
Many older people find their regular tasks challenging. Many people rely on outside aid to care for their aging relatives. Families all over the world are becoming increasingly concerned about elderly care. AI-powered in-home robots are the answer. These robots can assist the elderly with daily duties, allowing them to remain independent and at home, enhancing their overall health. One such example is ElliQ by Intuition Robotics. This is a virtual home assistant for older people to provide daily assistance, monitoring, and more.
Smart homes
Alarms and cameras with artificial intelligence (AI) are now at the forefront of cutting-edge home security. These security systems create a database of your home's frequent visitors using facial recognition software and machine learning models. This enables the system to recognize unwelcome visitors. Other intriguing capabilities include tracking when you last walked your dog or receiving notifications when your children return home from school. Some of the most recent systems can automatically dial 911, making it a viable option for subscription-based services in the same category.
Final words
In a word, machine learning applications are a significant advancement in the field of artificial intelligence. While machine learning has some unsettling implications, these machine learning applications are one of the ways that technology might help us live better lives.