How Does a Recommendation Engine Work With Predicting Likes?
•10 min read
- Languages, frameworks, tools, and trends
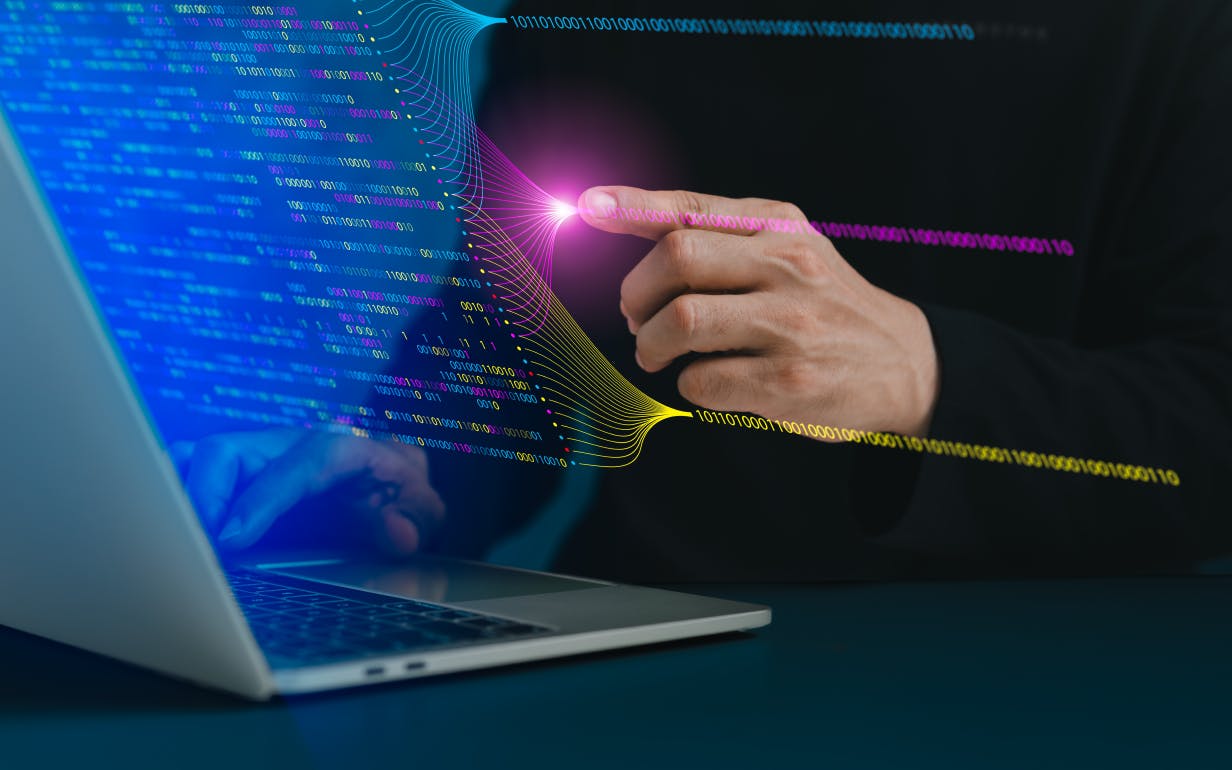
An automated recommendation engine suggests products and services to users based on machine learning. It is similar to a salesperson who knows what you like based on your history and preferences. Online recommendation engines strive to guide you toward the products you are most likely to purchase.
In today's digital world, recommendation engines are crucial as users are overwhelmed with options and need help finding what they want. The end result? Customers who are happier, which leads to more sales. This article will explore how a recommendation engine works, the types, and use cases.
What is a recommendation engine?
A recommendation system is a data filter tool that uses a machine learning algorithm to recommend the most relevant item for purchase to a customer. It is extensively used by businesses - especially in e-commerce, entertainment, mobile apps - as it enables them to provide personalized information or products to each customer.
The system operates on various factors containing customer behavior and user purchase history. The algorithm analyzes all collected data and makes a suggestion based on it.
Users rely on recommendation systems to understand the digital world via their experiences, behaviors, preferences, and interests. Any recommendation engine aims to keep users engaged and increase product demand.
The function of a recommendation engine is to populate multiple related products on website apps based on user data so as to enhance user experience. It can function in almost every business that provides personalized suggestions to users.
Popular examples of recommendation engines
Major companies like Amazon use recommendation systems to suggest products based on various marketing strategies. They include product recommendations for users, products that are frequently bought together, etc. The strategies also consist of off-site recommendations via email marketing.
YouTube uses a recommendation system to rank relevant videos, news, and channel subscription suggestions. It uses different data containing user interests, search history, likes and dislikes, and shares.
Social media platform, Facebook, uses a recommendation engine for friend suggestions and news feeds. It uses a system based on deep learning and neural networks called DLRM (deep-learning recommendation model), and also recommends groups or products on its Marketplace.
Entertainment giant, Netflix, uses a recommendation system for movie suggestions. In order to sort movies, the algorithm evaluates factors like user profiles (browsing history and ratings), movie types, trends, seasonality, and item-item similarities.
What is a product recommendation engine?
A product recommendation engine is a system combined with machine learning and artificial intelligence to generate product suggestions and predictive offers. The latter includes special deals and discounts on various products customized for customers.
An effective product recommendation engine uses customer behavior data analysis to create individual customer profiles. It uses these profiles to generate customized deals for specific customers who might be interested.
Types of recommendation engines
The following are the three types of recommendation engines:
Collaborative filtering
In a collaborative filter, the system focuses on data collection and analysis of user behavior. The data helps the system understand user preferences and predict that person's choice based on their similarities with other users. It uses a matrix-style formula to plot and calculate these similarities.
A collaborative filter chooses a product for a recommendation based on its user data analysis. It does not need to analyze raw content like products, videos, or books.
Content-based filtering
The working principle of content-based filtering is that if you like a particular product, you will choose a similar category product.
The system uses customer preferences and selected item descriptions like color and product type for a recommendation. The algorithm uses the cosine and Euclidean distance methods to calculate similarity.
The drawback of content-based filtering is that the system has limitations for recommending products. While it is capable of recommending items that a person has bought, it cannot recommend other products or content categories. For example, if a person buys kitchenware, the system can't recommend anything other than kitchenware.
Hybrid model
As the name suggests, a hybrid recommendation engine combines user behavior and content-based data. Using both data, it outperforms the other recommendation engines. Netflix is a leading example as it uses both user interests (collaborative data) and movie descriptions (content-based data).
Hybrid recommendation engines rely on natural language processing (NLP) to generate labels for each product and vector equations for similar product calculations. They use a collaborative filtering matrix to recommend products to users depending on their history, activities, behavior, and preferences.
How does a recommendation engine work?
A recommendation engine relies on combining datasets and the machine learning model. Data plays a significant role in the development of a recommendation engine as it helps the system to build different recommendation patterns. As the system collects and analyzes more data, it becomes more effective and efficient in making relevant revenue-generating suggestions.
Recommendation engines work on a four-step process: data collection, data storage, data analysis, and data filtering.
Step 1: Data collection
The process of building a recommendation engine starts with data collection. The system needs two types of data, implicit and explicit data.
Implicit data
This type of data includes information stored from user activities. It mainly contains web search history, cart events, click ratio, query searches, and order history.
Explicit data
This data contains information collected from customer inputs. It can include product reviews and ratings, likes and dislikes, and comments on the product.
Apart from this data, the recommendation system uses customer information, such as demographics (age, gender) and psychographic data (interest). This data helps the system categorize similar customers. It also uses content-based data (product genre, type) to identify and recommend similar products.
Step 2: Data storage
After data collection is complete, it's time to store that data. Note that as the system continues to work, it generates a lot more data. Scalable storage is, thus, necessary. There are various storage types (such as cloud, data servers, etc.) available depending on the data collected.
Step 3: Data analysis
Since the data collected and stored is in raw form, it must be sorted and analyzed before it can be used. There are different ways to analyze the data:
Real-time analysis
Data is analyzed as it is collected.
Batch analysis
Data is analyzed at regular intervals.
Near real-time analysis
Data is processed in minutes instead of seconds.
Step 4: Data filtering
This is the final step in the recommendation engine process. Different algorithms are used in the step depending upon the data. Once data filtering is complete, the recommendation is the outcome.
What is matrix factorization?
Complex operations on matrices can be calculated by breaking them into smaller parts using matrix decomposition. Also called matrix factorization methods, they are a fundamental part of linear algebra. They are used for mathematical operations, such as solving linear equation systems, inverse calculation, and calculating the determinant of a matrix.
The following example illustrates matrix factorization better. Below is the user-movie rating matrix (1-5) given by different users for different movies.
In the example, user_id is a unique ID for different users and movie_id is a unique ID assigned to movies.
We are trying to predict the missing ratings. A 0.0 rating represents that a particular user hasn't rated the movie. Here, 1.0 is the lowest rating a user can give to the movie. Matrix factorization can help us identify latent features that affect how a user rates a movie.
We will break down the matrix into small parts to ensure that the multiplication of these parts will generate the original matrix.
Now, we need to find k latent features. We will divide the rating matrix R(MxN) into P(MxK) and Q(NxK). Here, P x QT (in this case, QT represents the transposition of Q matrix) approximates the R matrix:
where:
- M represents the total number of users.
- N represents the total number of movies.
- K represents total latent features.
- R is the MxN user-movie rating matrix.
- P is the MxK user-feature A matrix representing the affinity between users and features.
- Q is the NxK matrix representing the correlation between movies and features.
- Σ is KxK, a diagonal matrix describing the weights of a feature.
With matrix factorization, latent features drop noise from data by removing the feature(s) that do not affect a user's rating.
To get ratings based on all latent features, we can calculate the dot product of the two vectors and add them together. This is how we can get a rating of rui for a movie qik rated by the user puk across all latent feature k:
As a result of matrix factorization, we can find ratings for movies that the users have not yet rated.
How can we predict likes with a recommendation engine?
A recommender system suggests similar items and ideas based on a user's specific way of thinking. In collaborative filtering, similar people tend to like similar things based on the data.
By analyzing the preferences of other similar users, it predicts which item a user will like. To generate recommendations, collaborative filtering uses a user-item matrix. The values in the matrix indicate how much a user prefers a certain item. The values can represent explicit user feedback (direct user ratings) or implicit feedback (for example, listening, buying, watching).
- Explicit feedback: User data collection depends on the amount of information they provide. The user usually chooses not to provide any data. It is, therefore, rare and sometimes expensive to obtain this data. For example, ratings from the user.
- Implicit feedback: Using implicit feedback, we predict user preferences based on their behavior.
For example:
Using a user x as an example, we need to find another user whose ratings are similar to x's rating. Then, we need to determine x's rating based on that other user's ratings.
The following matrix represents different users and movies:
With the matrix, we can represent different users and movies:
Imagine two users, x and y, with ratings rx and ry. Choosing a similarity matrix is the first step in calculating the similarity between sim(x,y).
There are several methods that can be used to calculate similarity: Jaccard similarity, cosine similarity, and Pearson similarity.
By subtracting the mean from the rating, we are using centered cosine similarity/Pearson similarity:
We can calculate similarity in this example: sim(A,B) = cos(rA, rB) = 0.09 ; sim(A,C) = -0.56. sim(A,B) > sim(A,C).
Rating predictions
The vector rx represents the rating of user x. Consider N to be a set of k similar users who rated item i as well. We can then use the following formula to predict the value of user x and item i:
Code section
In this section, we will be demonstrating how the recommendation is made based on the correlation between movies.
- Importing required libraries
import pandas as ps
- Importing required datasets
req_columns = ['user_id', 'item_id', 'rating', 'timestamp']
df_ratings = ps.read_csv(“ratings.csv”, sep='\t', names=req_columns)
df_movies = ps.read_csv(“movies.csv”)
- Merging the two datasets
df_merge = ps.merge(df_ratings, df_movies_titles, on='item_id')
- Calculating average of ratings for all movies
df_merge.groupby('title')['rating'].mean().sort_values(ascending=False).head()
Output:
- Creating a dataframe that consists of a “title”, “average rating”, “count of rating” per movie title.
count_df = ps.DataFrame(df_merge.groupby('title')['rating'].mean())
count_df['count of ratings'] = ps.DataFrame(df_merge.groupby('title')['rating'].count())
count_df.head()
- Sorting the movie title based on “count of ratings” attribute.
count_df.sort_values('count of ratings', ascending = False).head(10)
- Obtaining movies similar to the movie “Star Wars (1977)”
movie_pivot= df_merge.pivot_table(index ='user_id',
columns ='title', values ='rating')
starwars_1977_user_ratings = movie_pivot['Star Wars (1977)']
similar_to_starwars_1977 = movie_pivot.corrwith(starwars_1977_user_ratings)
corr_starwars_1977 = ps.DataFrame(similar_to_starwars_1977, columns =['Correlation'])
corr_starwars_1977.dropna(inplace = True)
corr_starwars_1977.head()
- Similarly, we can obtain movies similar to others based on their correlation.
Use case of recommendation systems
Here are a couple of use cases of recommendation engines from a well-known organization.
Audio streaming platforms
It can be challenging to choose just one song from an entire collection of songs in different genres on audio streaming platforms. This can be solved through AI-enabled recommendations. They are embedded in smart audio streaming platforms that monitor customer listening patterns.
Based on individual preferences, the system provides customers with personalized playlists that they are most likely to listen to in the upcoming weeks and months.
Example: Spotify
Music streaming giant, Spotify, uses artificial intelligence to regularly update users' weekly discovery playlists to keep them informed of the latest tracks by their favorite artists.
The company has also acquired music intelligence and data platform, The Echo Nest, which offers concerts, software analysis, and NLP to generate a music recommendation engine based on three different models, including collaborative filtering and audio file analysis.
We’ve seen how recommendation engines work and learned how to build one with matrix factorization. We’ve also discussed a few use cases. Recommendation engines are a great way of keeping an e-commerce platform fresh. If you want to add more products and increase sales, the best way to do so is to display products customers will be attracted to. These systems are used in many ways and they're becoming even more popular among businesses.
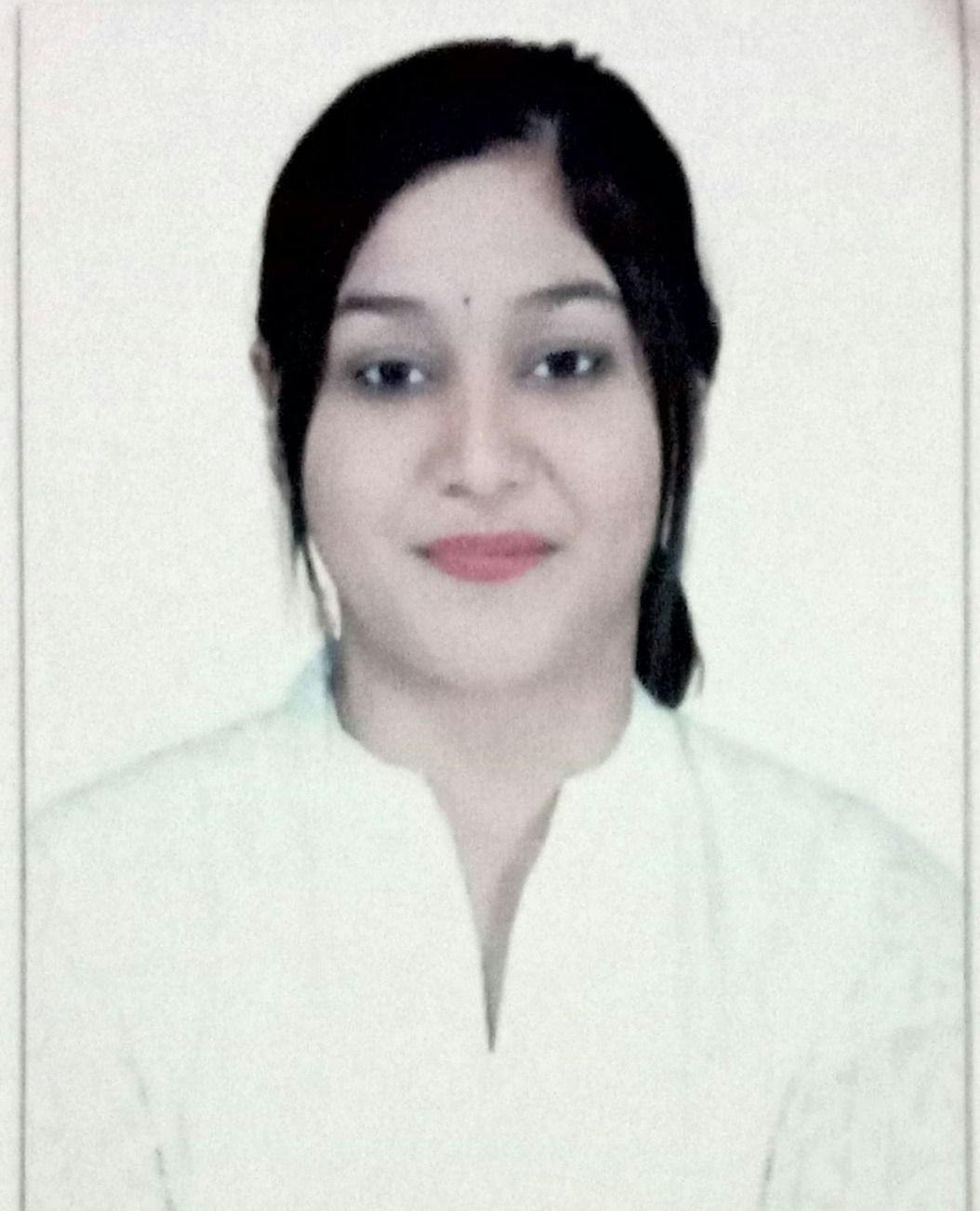
Author
Sanskriti Singh
Sanskriti is a tech writer and a freelance data scientist. She has rich experience into writing technical content and also finds interest in writing content related to mental health, productivity and self improvement.