Hadoop Ecosystem: Hadoop Tools for Crunching Big Data Problems
•16 min read
- Languages, frameworks, tools, and trends
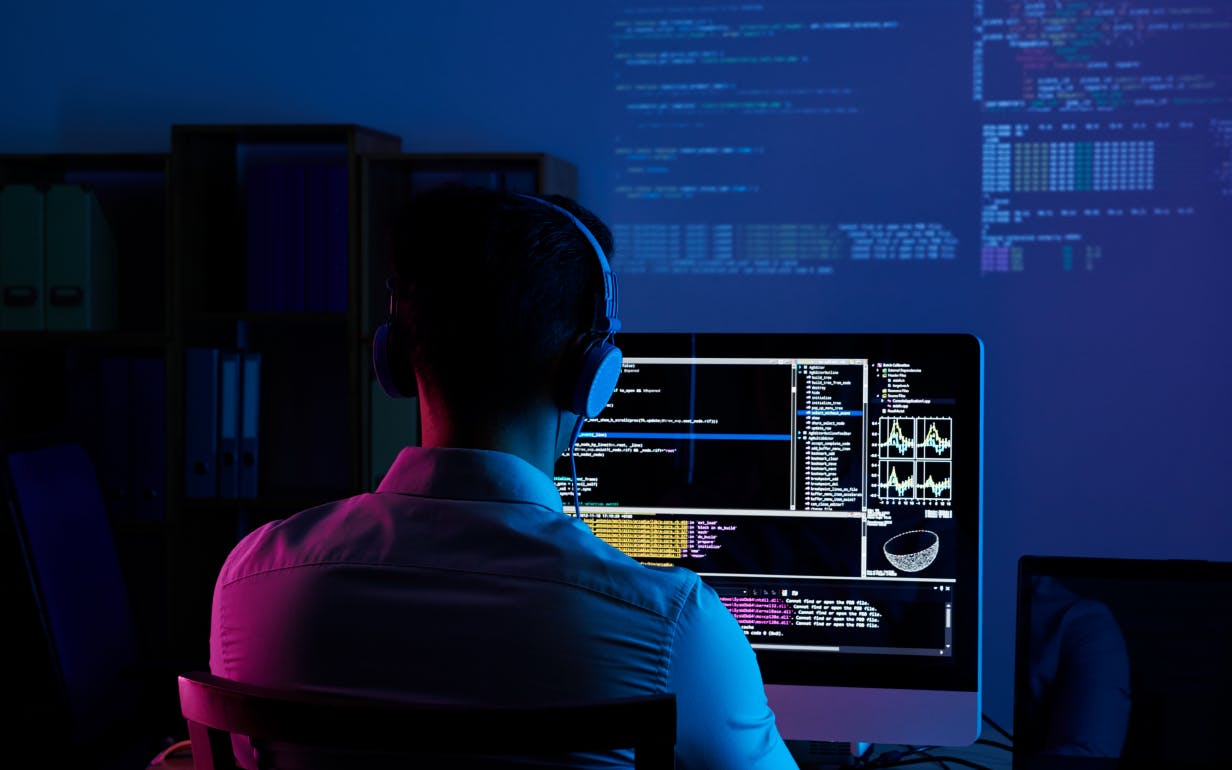
Big Data has been a sensation in the IT industry since 2008. Data generation is huge in the current technological world. Many different sectors like telecom, social media, health care, insurance, manufacturing, stocks, and many more are generating a huge amount of data.
Before the emergence of Hadoop, processing and storing large volumes of data was challenging. But with the help of Hadoop and the Big Data ecosystem, industries have been blessed in understanding large data volumes and driving themselves towards growth.
Here are some instances where you will find Apache Big Data Ecosystem very helpful:
- Costly machines for quality testing are not enough, but capturing data and analyzing it would reveal patterns for improvement.
- Capturing data and analyzing it will also help you in determining your customer’s interests.
- Stock markets have tons of data, which will correlate every time and with a Big Data ecosystem, you can get great insights.
- Forecasting any product for the future is made easy.
Many useful insights on applications have been found with the emergence of the Big Data ecosystem. Hadoop machine learning is the answer to the dwelling problem of analyzing large amounts of data. The Hadoop Big Data ecosystem is a combination of different technologies which has proficiency in solving data-related problems.
Now, let’s understand the Hadoop Ecosystem components in the Big Data ecosystem to create the right solution for any data-related problems of our business.
Hadoop Ecosystem
Organizations have been using traditional systems like data warehouses and relational databases for decades to analyze and store their data. But the data generated today are too huge for those systems to handle.
More semi-structured or unstructured data are usually generated nowadays and the previously used systems were meant to handle structured data. Those systems were vertically scalable which required adding more storage, processor, and memory to the system which made it expensive.
Also, the data from today are stored in different silos, which together when analyzed, will help you in finding a pattern that might not be possible with those machines. This is where the Hadoop Ecosystem comes in and turns all your problems into successful solutions.
The Hadoop Ecosystem is a platform that will give you different services for solving your data problems. The Hadoop components include Apache projects and other commercial tools for finding a perfect solution.
All these tools will work together to collectively provide services like analysis, absorption, storage, and maintenance of your data. If you want to understand the Hadoop Ecosystem, the following diagram and this article will guide you through it.
These changes have made the organization support the Hadoop Ecosystem massively. Now, organizations have started hiring more Hadoop developers who are knowledgeable in this platform for improving their customer experience and solving their big data challenges.
What is Hadoop?
Many big companies have faced the above-mentioned challenges when they wanted to analyze and store their data. Relational databases were too costly to maintain and not flexible enough, that’s when the use of Big Data started emerging.
Apache Hadoop is an open-source framework that deals with large volumes of data in a distributed environment. It is designed based on the Google File System which was created by Google. This environment is built of different machines which will work together for providing a single machine.
Some vital properties of the Hadoop you should understand are as below:
- When compared to vertical scaling in RDBMS, the horizontal scaling of Hadoop provides a higher impact.
- It is highly scalable as it handles data in a distributed manner.
- It will utilize the data locality concept for processing the data on the nodes on which they are stored rather than moving it over the network reducing the traffic.
- It will create and store replicas of data making and it is fault-tolerant.
- It will be able to handle any data type.
- It is cost-effective as all nodes are in clusters which is not expensive.
Components of the Hadoop Ecosystem
The following Hadoop components in Big Data will help in understanding the ecosystem better and also help you handle your data efficiently.
Core Hadoop
HDFS, YARN, and Map Reducer are the core components of the Hadoop Ecosystem. We will discuss them in detail below.
HDFS
Hadoop Distributed File System is the backbone or core component of the Hadoop Ecosystem. HDFS helps store structured, unstructured, and semi-structured data in large amounts. It works as a single unit, as HDFS creates an abstraction over the resources.
HDFS maintains the log files about the metadata. The files in HDFS break block-sized into chunks. Each file is divided into blocks of 128MB (configurable) and stored on different machines in the cluster.
HDFS master/slave architecture
This architecture has two main components NameNode and DataNode. A single NameNode works as a master and multiple DataNodes perform the role of a slave. Both NameNode and DataNode are capable enough to run on entity machines.
Name node
The NameNode is the master node that maintains and manages the blocks present in the Data nodes. The NameNode doesn't store the actual data. It contains metadata, just like a log file or a table of content. Therefore, it requires less storage and high computational resources.
It is a duty to know where each of the blocks belonging to a file is fibbing in the cluster. HDFS handles the file system namespace by performing an operation like opening, renaming, and closing the files. As it is a single node, it may evolve the cause for single-point failure.
Data node
DataNode is a slave node and all data are stored in it, hence it requires more storage resources. The DataNodes are the hardware like laptops and desktops in the distributed environment. And that makes Hadoop solutions cost-effective. Its task is to retrieve the data as and when required.
YARN
YARN or Yet Another Resource Manager serves all processing activities by allocating resources and scheduling tasks. YARN allows data stored in HDFS to be processed and run by different data processing engines such as batch processing, stream processing, interactive processing, graph processing, and more.
Following are the components of YARN:
- ResourceManager - It is used in the processing department to receive the processing requests, and then gives the parts of requests to connected NodeManagers consequently, where the actual data processing takes place.
- NodeManagers - These are installed on every DataNode. It is responsible for executing the tasks on every single DataNode.
- Schedulers - According to application resource requirements, Schedulers conduct scheduling algorithms and allocate the resources to the application.
- ApplicationsManager - These will simply accept the job submission, negotiates to containers for executing the application-specific, and monitors the progress.
- ApplicationMasters - These are the daemons that live on DataNode and communicate to containers to execute tasks on each DataNode.
MapReduce
To handle Big Data, Hadoop relies on the MapReduce algorithm introduced by Google in 2004. MapReduce is the core component of the Hadoop Ecosystem that helps in processing, which means it provides the logic of processing.
In simple words, MapReduce is a software framework of distributed and parallel algorithms inside the Hadoop environment that helps to write applications that process large data sets. MapReduce works in a divide-and-conquer pattern.
To handle the huge amount of data in a parallel and distributed form, the data follows a particular flow from various phases:
- Input reader - The task of the input reader is to read the incoming data. The input data can be in any form. It then splits the data into the data blocks of the fitting size of 64 MB to 128 MB as per the requirement. Every data block is associated with a Map function (). Once the input reader reads the data, it generates the corresponding <key, value> pairs, and the keys will not be unique in this case.
- Map function - The map function () helps to process the coming pairs and generates the corresponding output pairs. The map function executes actions like sorting, filtering, and grouping.
- Partition function - The partition function helps to assign the output of each map function to the fitting reducer and returns the index of reducers.
- Shuffling and sorting - The data is shuffled between/within nodes, and it rolls out from the map to get ready and process further reduced functions. The sorting operation is performed on input data for the Reduce function. Here, the data comparison happens, using a comparison function, and then is sorted.
- Reduce function - Reduce function aggregates and summarizes the result. The Reduce function assigns each unique key and these keys were already arranged in sorted order. The values associated with the keys can iterate the Reduce and generate the corresponding output.
- Output writer - The Output writer is executed once the data flow from all the above phases. The main task of the Output writer is to write the Reduce output to the stable storage.
Data Access
There are several data access tools in a Hadoop Ecosystem, you can start by using the following tools:
Apache Pig
The pig was developed to analyze large datasets. Pig helps to overcome any difficulty of writing maps and reducing functions. And it has two parts:
- Pig Latin - It is a language.
- Runtime - The runtime for executing the code in any environment.
Internally, the code written in Pig converts into MapReduce functions and helps programmers (familiar with Java).
The compiler in Pig will convert Pig Latin code into MapReduce internally. It will produce a sequential set of jobs that are an abstraction. It was developed by Yahoo and it will provide you with a platform to build data flow for ETL, analyzing, and processing large datasets.
Apache Hive
Apache Hive is a distributed data warehouse system developed by Facebook and is used to analyze structured data. Hive is built on top of Hadoop and operates on the server side of a cluster. The hive is commonly used by Data Analysts to create reports.
Hive help to perform reading, writing, and managing large data sets in a distributed environment using a SQL-like interface. Hive supports Data Definition Language (DDL), Data Manipulation Language (DML), and User-Defined Functions (UDF). Hive is not capable of handling real-time data and online transaction processing. It has two components:
- Hive Command-Line interface runs HQL commands Java Database Connectivity (JDBC)
- Object Database Connectivity (ODBC) driver establishes connections from data storage.
Data Storage
HBase and Cassandra are the two popularly used tools in the Hadoop Ecosystem for data storage. Here is more about them.
HBase
HBase is a column-based NoSQL database and runs on top of HDFS to handle any kind of data. HBase allows for real-time processing. The HBase is written in Java, and its applications can be written in REST, Avro, and Thrift APIs.
It will support different data types and it will be capable of handling the entire Hadoop Ecosystem. It is modeled after Google’s BigTable which is a distributed storage system for coping with larger datasets.
Cassandra
A NoSQL database that is designed for high availability and linear scalability is Cassandra. It is based on the key-value model and it is developed by Facebook. It provides swift responses to queries. It has column indexes and supports de-normalization. It will have materialized views and is influential in caching.
Interaction - execution & development
Execution and development of the Hadoop Ecosystem in Big Data are done with the following tools.
Hcatalog
A table management layer that provides integration of hive metadata for other Hadoop technologies. It allows the user with various processing tools to read and write data easily. It will provide a tabular view of various formats and provide data availability of different notifications. The REST APIs for the external system will help you in accessing metadata.
Apache Crunch
Apache Crunch is built to pipeline MapReduce programs that are efficient and simple. This framework is used to write, test, and running MapReduce pipelines. It is developer-focused, offers a flexible data model, and has minimal abstractions.
Apache Hama
A distributed framework that depends on BSP (Bulk Synchronous Parallel) computing is known as Apache Hama. It is capable of massive computations like graph, network, and matrix algorithms. It is a well-suited iterated algorithm that supports YARN. Hama will provide collaborative filtering unsupervised machine learning applications.
Apache Solr & Lucene
Apache Solr and Apache Lucene are two different services. The relationship between both is that of a car and its engine. We are incapable of driving an engine, but we can drive a car. Similarly, Lucene is a programmatic library that may not use as-is, whereas Solr is a complete application that you can use out-of-box.
Data Intelligence
The Hadoop tools for understanding data intelligence are explained below:
Apache Drill
Apache Drill is an open-source application for analyzing larger datasets in distributed environments. It will support various NoSQL databases. And will follow the ANSI SQL combining different kinds of data stores in one query.
The major reason for this is providing scalability that will help you in processing petabytes and exabytes of data very effectively. It is a replica of the Google Dremel. Apache Drill has a very powerful scalability factor that supports many users by serving their query requests over large-scale data.
Apache Mahout
Apache Mahout is an open-source project, that provides an environment for creating machine learning applications that are scalable. Apache Mahout performs recommendation, classification, and clustering machine learning techniques.
Machine learning algorithms allow you to build self-learning machines that will evolve around themselves, which are being programmed explicitly. According to user behaviors, past performances, and data patterns, it will make vital decisions. You can also name it AI.
Mahout performs a collaborative filter, classification, and cluster protocols which are explained below:
- Collaborative filter - It will mimic user behaviors, and their characteristics, and the data patterns accordingly will predict what the users must do.
- Collaborative classification - It will classify and categorize the data into different sub-departments and categories.
- Collaborative cluster - It will help you with organizing similar data groups.
- Missing frequent item set - The Mahout will check objects that might appear together and suggest if anything goes missing.
Mahout will provide a command line that will invoke different algorithms. It will have a predefined library that contains different inbuilt algorithms for various use cases.
Apache Spark
Apache Spark is used for real-time data analytics in a distributed computing environment. It is written in Scala and supports many different languages. Apache Spark is 100x faster than MapReduce when processing large-scale data as it will perform in-memory computations and optimizations.
Also, Apache Spark Framework has its ecosystem with the following components:
- Spark core - It will act as an execution engine for Spark and other APIs that are built on top of it.
- Streaming API - It will enable Spark to handle real-time data and integrate it with other data sources.
- MLlib - It is a scalable machine learning library that enables Data Science tasks.
- Spark SQL API - It stores query structured data in DataFrames.
- GraphX - It is a graph computation engine that works with the ecosystem.
Serialization
Apache Avro and Apache Thrift are some essential Hadoop tools for performing data serialization.
Apache Avro
A data serialization framework that is language neutral is known as Apache Avro. It is designed for performing across languages which allow data to potentially outlive the language for reading and writing it.
Apache Thrift
A language developed for building interfaces that interact with the Hadoop-built technologies is Apache Thrift. It is used for defining and creating services for many different languages.
Integration
Some Hadoop Ecosystem tools that will help you with data integration:
Apache Chukwa
Apache Chukwa is an open-source data collection system that will help you in monitoring larger distributed systems. It is built on the HDFS and MapReduce framework. It inherited its robustness and scalability from the Hadoop Ecosystem.
It includes a powerful and flexible tool for monitoring, analyzing, and displaying results and making the best use of the collected data. The Kafka routing service plays a major role in moving data from Kafka to different sinks.
Apache Samza is used for this routing. When Apache Chukwa sends its traffic to Kafka, it delivers full streams or filtered ones as per our request. Sometimes, we should apply many filters to the streams which will be written using Chukwa. The router should perform one Kafka topic for producing a new Kafka.
Apache Sqoop
Apache Sqoop is a structured data ingesting service that can import and export structured data from RDBMS or Enterprise data warehouses to HDFS or vice versa. It performs with nearly all relational databases like MySQL, Postgres, SQLite, and more.
When we submit the Sqoop command, the main task converts internally into MapReduce tasks which are then executed over HDFS. Together, all the Map tasks import the whole data. When we submit our job, it is mapped and will bring the chuck of data from HDFS. These chunks will then be exported to a structured destination.
Apache Flume
Intake of the data is a vital part of the Hadoop Ecosystem and Apache Flume plays a major role in it. It is a service that will help you with the process of intaking unstructured data into HDFS. It will also provide a solution that is distributed and reliable.
It will also help you with aggregating, moving, and collecting large datasets. It will also help you with the online streaming of data from different sources into HDFS. A Flume agent will ingest the streaming data from various sources to HDFS.
There are three main components of the Flume agent as follows:
- Source - It will accept the data from the streamline and will store it in the channel.
- Channel - It will act as local storage. It is temporary storage between the data source and the persistent data in the HDFS.
- Sink - Sink will collect the data from the channel and will write it permanently to the HDFS.
Management & Support
Here are some of the Hadoop tools that will help you with support and management of your Hadoop Ecosystem:
Apache Zookeeper
Apache Zookeeper is an open-source that coordinates with various services in a distributed environment. It was challenging and time-consuming to coordinate between different services in the Hadoop Ecosystem.
To solve these problems, Zookeeper was introduced. Zookeeper saves up time by following the process of synchronization, configuration maintenance, grouping, and naming. The Apache Zookeeper is a replica of the Google Dremel.
It will also support different kinds of NoSQL databases and filesystems similar to the Apache Drill. Rackspace, eBay, and Yahoo are some of the bigshots which are using Apache Zookeeper and are benefiting from it.
Apache Oozie
Oozie is a workflow scheduler to schedule Hadoop jobs and bind these together as one logical work. Oozie helps in job scheduling in advance and creating a pipeline of individual jobs to be executed sequentially or in parallel to achieve a sizable task.
Apache Oozie jobs are of two types:
- Workflow - The Apache Oozie Workflow is a set of actions that need to be performed. It is performed like a relay race where one task is completed to proceed to the next.
- Coordinator - These jobs will trigger when the data is available in it. It is a response-stimuli system that will answer when there is a new task available, else will be idle.
Apache Ambari
Apache Ambari is an open-source administration tool deployed on top of the Hadoop cluster and is responsible for keeping track of running applications and their status. In other words, consider an open-source web-based management tool that manages, monitors, and provisions the health of Hadoop clusters.
Apache Ambari will provide:
- Provisioning - It will provide a step-by-step installing process for your Hadoop services across different hosts. It will also handle the configuration of Hadoop services in a cluster.
- Management - It will give the central management system to start, re-configure, or stop a Hadoop service across the cluster.
- Monitoring - It will help you with a dashboard for monitoring the health and status of your clusters. It has an Amber Alert Framework which will notify the user when there is an emergency.
Wrapping up
Organizations can consider using the Hadoop tools for solving most of their Big Data problems. Hadoop is an open-source platform that can be used if you have the necessary skills. Also, Hadoop is a highly scalable and easy-to-use software that doesn’t require you to invest a huge amount in the infrastructure.
The success of the Hadoop Ecosystem is mainly because of the developer communities that contributed to increasing its productivity. However, inside the Hadoop Ecosystem, understanding one tool will not help you in coming up with the perfect solution, you need lots of tools to resolve your Big Data problems, so when you want to achieve high success ensure you read a set of relatable and needful tools of all types to make use of it.
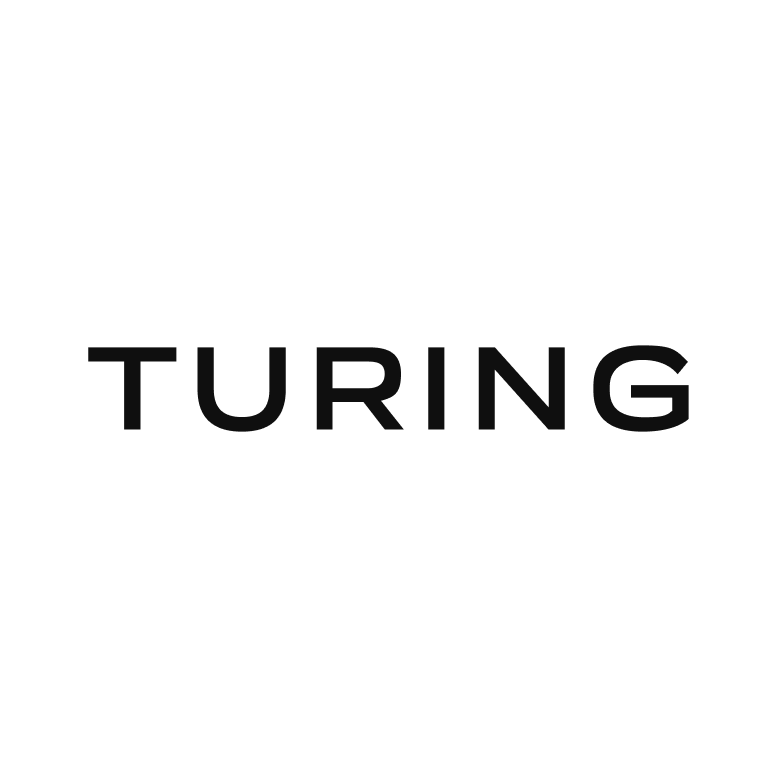
Author
Turing Staff