Best Strategies to Minimize Hallucinations in LLMs: A Comprehensive Guide
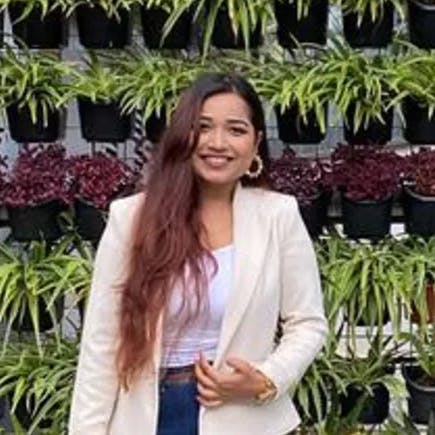
Ambika Choudhury
•8 min read
- LLM training and enhancement
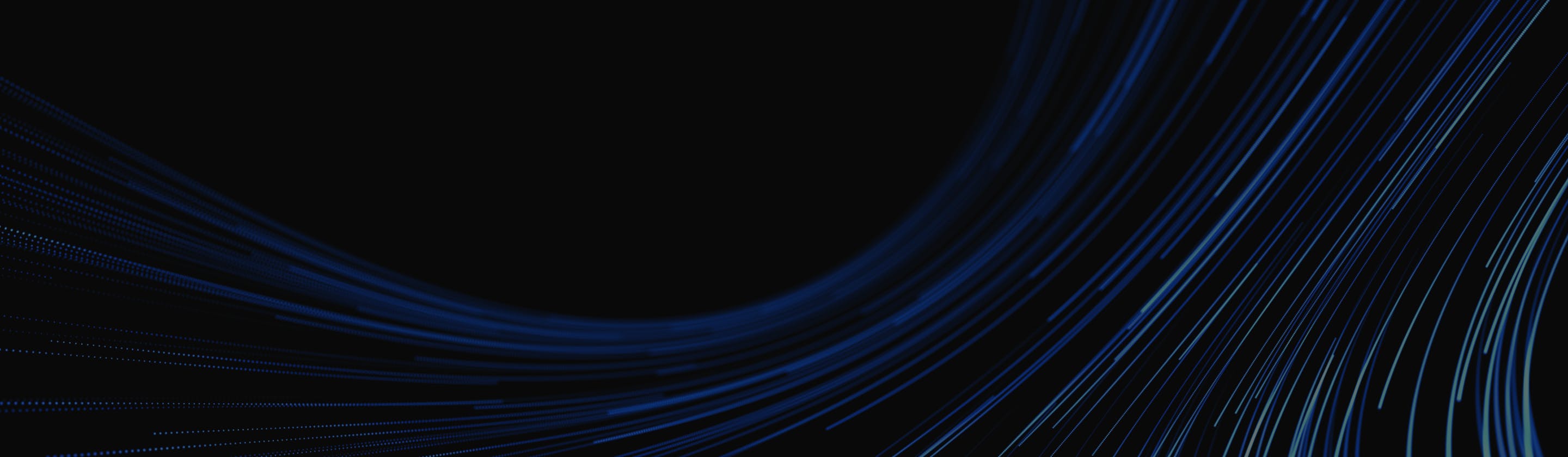
In the rapidly evolving landscape of large language models (LLMs), achieving high levels of accuracy and coherence is crucial. However, despite significant breakthroughs in natural language processing and machine learning, "hallucinations" continue to be a key challenge with LLMs. According to reports from January 2024, the occurrence of hallucinations in publicly available LLMs spans roughly between 3 and 16 percent. These hallucinations range from inaccuracies to factual errors, potentially undermining the credibility and utility of LLM applications across various industries.
This comprehensive guide seeks to demystify LLM hallucinations by providing a thorough understanding of what they are, their types, and real-world examples that highlight their impact. Further, we delve into the reasons behind these hallucinations, dissecting their common causes and exploring the neural mechanisms that lead to such fascinating yet problematic outputs. We also discuss the future directions in model training and architecture improvements that aim to mitigate hallucinatory outputs while enhancing model robustness and reliability.
Understanding hallucinations in LLMs
LLM hallucinations occur when models generate incorrect or nonsensical information, and they can pose significant challenges to the model’s credibility and reliability. Before delving deep into the strategies for reducing hallucinations in LLMs, let’s look at the fundamentals first.
What are LLM hallucinations?
LLM hallucinations refer to instances where LLMs generate information that is incorrect, nonsensically structured, or unrelated to the input provided. This information can vary from minor errors to larger issues like fabrications and distortions of facts, frequently leading to user confusion or creating a misleading impression of the models' comprehension and output capabilities.
In contrast to human mistakes, which usually come from misunderstandings or gaps in knowledge, hallucinations in LLMs emerge from the inherent functions and limitations of the models themselves. For instance, LLMs like the GPT (Generative Pre-trained Transformer) series, BERT (Bidirectional Encoder Representations from Transformers), and their derivatives learn to predict the next word in a sequence based on vast amounts of text data they have been trained on. They don't "understand" the content in the human sense but rely on statistical patterns and relationships between words to generate plausible-sounding text based on the inputs they receive.
Types of LLM hallucinations
In recent research, LLM hallucinations have been categorized into three types.
Fact-conflicting hallucination
Fact-conflicting hallucinations happen when LLMs create information or text that doesn't match what is known to be true. There can be many reasons for these errors, which can happen at any time during the different stages of the LLM life cycle.
Input-conflicting hallucination
Input-conflicting hallucinations occur when the output produced by an LLM diverges from what the user specifies. Generally, the user's input into an LLM consists of two parts: the task direction (for instance, a user prompt for summarization) and the task material (for instance, the document to be summarized). A discrepancy between the LLM's output and the task direction often indicates a misinterpretation of the user's objectives.
On the other hand, when the divergence is between the generated content and the task material, the hallucination aligns with the traditional understanding of the term in specific natural language generation (NLG) tasks like machine translation and text summarization.
Context-conflicting hallucination
In context-conflicting hallucination, LLMs may produce outputs that are inconsistent or contain self-contradictions, something particularly noticeable in longer or multipart responses. These hallucinations occur when LLMs either overlook the broader context or have difficulty maintaining uniformity throughout an exchange. Such issues lie in the models' inherent limitations in retaining context awareness over extended interactions or identifying the context required for maintaining coherence.
Why do LLMs hallucinate?
In 2023, Google announced its popular LLM chatbot Bard. A GIF from Google showed Bard answering a question from a 9-year-old about what cool things the James Webb Space Telescope found. Bard mistakenly said the telescope took the first-ever pictures of a planet outside our solar system. But actually, that wasn't done by the James Webb Telescope. The real first pictures of planets far away in space were taken by the European Southern Observatory's Very Large Telescope back in 2004, something NASA confirms as true.
LLMs generate misleading, unrelated, or factually incorrect outputs due to the nature of their training and inherent limitations. These models are statistical systems that learn from vast amounts of text data and identify patterns to predict the next word in a sequence without true understanding or awareness of facts. Their knowledge is based on the data they are trained on, which can contain inaccuracies, biases, and misinformation, which can lead these models to replicate or amplify such issues.
The way LLMs are designed prioritizes fluency and coherence over factual accuracy, causing them to produce plausible-sounding but entirely fabricated statements especially when faced with ambiguous prompts or incomplete information.
Furthermore, because these models' training data are frozen after a certain time, they cannot incorporate new information or correct errors without retraining, further contributing to the generation of incorrect outputs.
Your AI might sound convincing, But is it getting the facts right?
Fix It Now.How are LLM hallucinations detected?
Detecting hallucinations in LLMs involves various approaches that combine automated tools, validation against factual databases, and human judgment. One method to detect hallucinations is by cross-referencing LLM-generated content with trusted and authoritative sources. This could be structured databases for factual information, reputable news outlets for current events, or peer-reviewed journals for scientific claims.
Also, out-of-distribution (OOD) detection techniques can identify when a model is generating content based on inputs or contexts that it’s less certain about, which might lead to potential hallucinations. By analyzing the model's confidence levels or using dedicated OOD detection models, one can flag outputs likely to be inaccurate or fabrications.
Strategies to reduce LLM hallucinations
Let’s look at some of the key strategies that can be used to reduce LLM hallucinations:
Advanced prompting methods
Advanced prompting techniques are strategic approaches to querying LLMs that enhance the model's understanding of the task and guide it toward generating more accurate, relevant, and coherent responses.
One such technique is chain-of-thought prompting, which encourages the model to break down its reasoning into intermediate steps before arriving at a final answer. This method is particularly useful for complex problem-solving or explanatory tasks. By forcing the model to articulate a clear reasoning path, chain-of-thought prompts can reduce errors or hallucinations that might occur if the model were to jump directly to a conclusion without showing its work.
Another popular technique is the few-shot prompting. In few-shot prompting, the inclusion of a few carefully selected examples within the prompt acts as a guide for the model. These examples demonstrate the kind of output that's expected, helping the model to replicate this format, style, or level of detail in its responses. By showing the model what correct responses look like, few-shot prompts can significantly narrow down the model's focus and encourage it to produce similar, factually grounded content.
Retrieval-augmented generation (RAG)
Retrieval-augmented generation (RAG) is a methodology that combines the strengths of information retrieval methods with the generative capabilities of LLMs to produce more accurate and contextually relevant outputs. This approach can effectively reduce the incidence of hallucinations by generating plausible but factually incorrect information in LLMs.
Despite RAG’s role in mitigating hallucinations, challenges persist as LLMs can still produce content that misaligns with or outright contradicts the information retrieved during the augmentation process. To effectively address and minimize hallucinations within the framework of RAG, it becomes imperative to establish benchmark datasets specifically designed to quantify the extent of hallucination. This is crucial for developing more sophisticated strategies for hallucination prevention. A promising method to evaluate and consequently improve the performance of LLMs in minimizing hallucinations involves the utilization of specialized tools such as the Retrieval-Augmented Generation Benchmark (RGB) and RAGTruth.
The Retrieval-Augmented Generation Benchmark (RGB) is a unique data set specifically created for testing RAG across the English and Chinese languages. It sets itself apart by organizing the data into four unique testbeds focused on the key skills needed to overcome the issue of hallucinations, thus offering an all-encompassing method to evaluate the efficacy of LLMs. A study conducted on six distinct LLMs with the application of RGB was designed to uncover common challenges faced when implementing RAG. The results from this study revealed that despite LLMs showing a certain level of tolerance to noisy data, they significantly struggle with rejecting false information (negative rejection), synthesizing data effectively (information integration), and identifying incorrect information.
RAGTruth represents another focused collection designed to closely examine hallucinations at the word level, spanning numerous domains and activities within the conventional RAG setups for LLM usage. Gathering almost 18,000 authentic responses generated by different LLMs employing RAG, this corpus marks a substantial effort in comprehending and reducing the occurrence of word-level hallucinations.
Few-shot and zero-shot learning
Few-shot and zero-shot learning can allow LLMs to generalize effectively from limited examples or even in the absence of explicit examples for a specific task. Few-shot and zero-shot learning not only enhance the models' ability to generalize from minimal examples but also play a significant role in mitigating the occurrence of hallucinations.
When employing few-shot learning, LLMs are given a handful of examples from which to learn that help them understand the context and desired output format more precisely, thus minimizing the chances of generating contextually irrelevant or factually incorrect (hallucinated) information.
Zero-shot learning, on the other hand, enables LLMs to infer the correct response to tasks they haven't been explicitly trained on by relying on their broad understanding of language and knowledge patterns, which can prevent the model from making unsupported assumptions or fabrications.
Fine-tuning LLMs
Fine-tuning adjusts the LLM's learned patterns to closely align with the nuances, vocabulary, and factual information specific to the new context to improve its accuracy and relevance. By training the LLM on a curated set of data, it can correct or update its knowledge base, thus mitigating the propagation of errors or outdated information inherent in its broader pretraining.
Moreover, fine-tuning enhances the model's ability to generate responses that are not only factually correct but also contextual and coherent within the specific domain, further reducing the likelihood of generating hallucinated content.
Conclusion
Minimizing hallucinations in LLMs is vital for enhancing their dependability and extending their utility across various sectors. The strategies discussed outline a multipronged approach to confronting this challenge effectively. Each strategy offers distinct advantages in enhancing model understanding, factual accuracy, and the continual relevance of information.
The LLM training and development services experts at Turing tackle this issue through a combination of deep technical knowledge, cutting-edge research, and practical application. They employ advanced techniques, such as fine-tuning the models with curated, high-quality datasets to correct inaccuracies and implementing sophisticated algorithms that detect and reduce hallucinations. Additionally, they leverage their vast experience to develop innovative strategies. By continuously updating the models with the latest information and insights, Turing's experts ensure that the LLMs not only produce reliable outputs but also remain at the forefront of AI technology.
Still getting made-up answers from your AI?
Upgrade your LLM’s performance with targeted fine-tuning and continuous evaluation from the leaders in model training.
Optimize Your LLM Today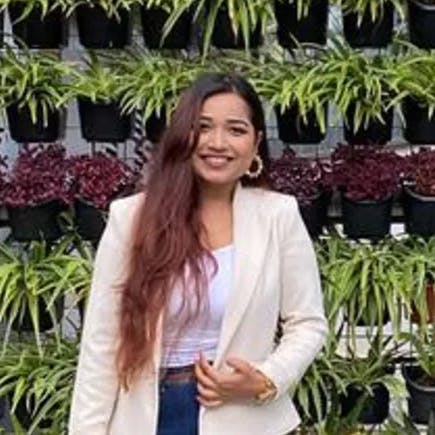
Author
Ambika Choudhury
Ambika is a tech enthusiast who, in her years as a seasoned writer, has honed her skill for crafting insightful and engaging articles about emerging technologies.