How to Reduce Bias in Artificial Intelligence
•7 min read
- Languages, frameworks, tools, and trends
- Skills, interviews, and jobs
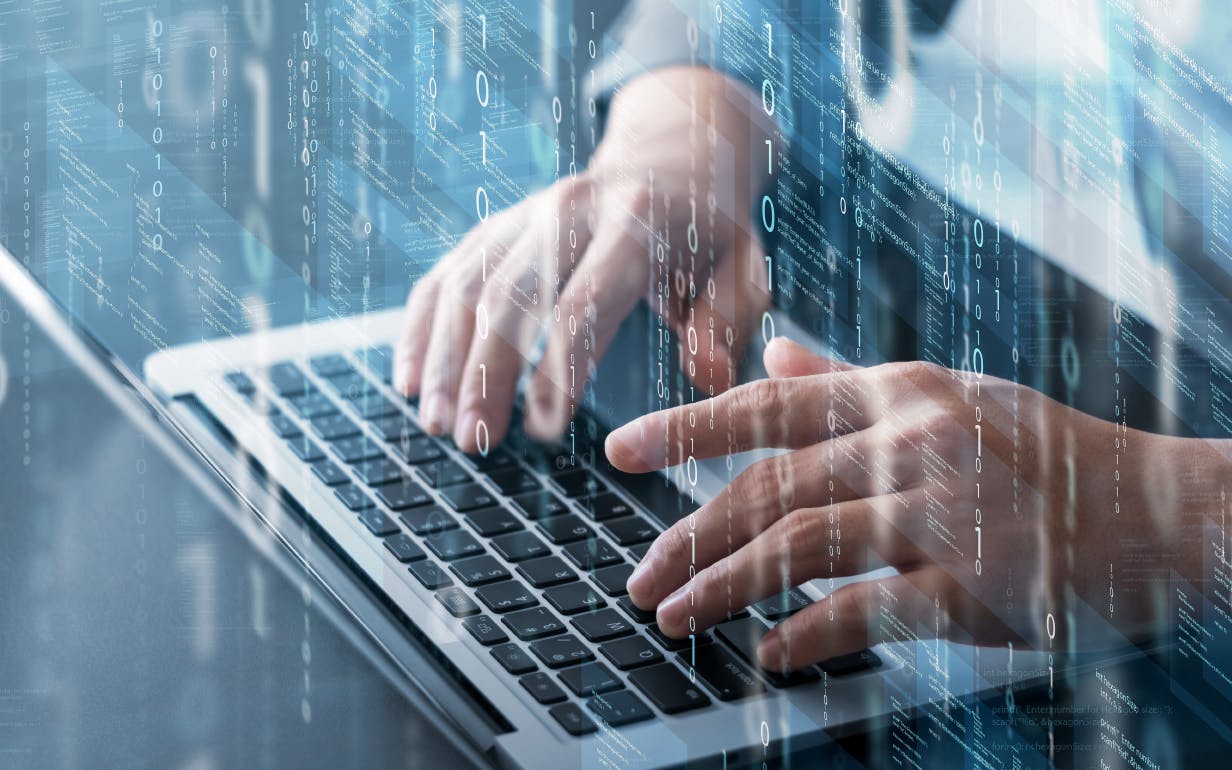
It may take years to eradicate human bias, but preventing bias in AI isn’t a daunting task. AI beholds the power of working fairly compared to humans. However, much evidence lately has illustrated that AI models can embed societal and human biases and deploy them on a scale.
Human creators have unwillingly and unintentionally induced bias into the systems by training the model on biased data or with rules that were created by experts with implicit biases.
It’s important to eliminate it for some crucial reasons listed below.
- It helps decision-making systems less prone to bias and ultimately offers equality and justice.
- It also helps in delivering the right marketing messages from a brand to its potential customers and others.
In this article, we will discuss AI bias and how you can reduce its risk in your AI models for fair and square results.
AI bias: Algorithm reflecting human bias
AI bias, algorithm bias, or machine learning bias is a phenomenon in a machine learning process where the algorithm offers systematically biased results based on some erroneous assumptions. It has been commonly reported in the news that headlines were not able to identify the pronouns correctly, or a failed face recognition software that recognizes people of a certain color only.
However, the bottom line is that the experts write and train these machine learning models on socially generated data. In today's context, it can pose a risk and a challenge of inducing bias into these models. The samples of training data are not compared, validated, and monitored for outliers by applying statistics and data exploration.
In the next segment, we will get you acquainted with some examples of AI bias that will help you visualize the big picture.
Examples of AI bias
Check out the below-mentioned common examples of AI bias that are sure to amaze you.
The hiring algorithm of Amazon
Amazon is the dominator of the e-commerce industry. It manages its empire by putting tasks to automation like making price changes or making changes in the warehouse operations.
However, it is claimed by some individuals that the company leverages AI recruiting tools to assign job applicants ratings, which much similar to how consumers rate products on Amazon. Following these claims, Amazon discovered that the rating was not being done in a gender-neutral manner and they made the required changes.
What went wrong?
Amazon computer models were analyzing candidate resumes for decades which made it realize the male dominance in the industry. When the Amazon algorithm learned about it, it eliminated resumes that belonged to a female candidate. Moreover, the applications of those female candidates who attended one of two all-female institutions were also demoted in the process.
How was the bias reduced?
This bias was fixed by Amazon by changing the programs. But, it doesn’t mean that the bias that wasn’t claimed or found was also eliminated.
Racial bias in the healthcare system of America
Every human being has an equal right to quality healthcare. But, the AI systems trained on non-representative data in the American healthcare system narrate a different story for the underrepresented population.
It was found in 2019 that the algorithm used in US hospitals favored white patients over black patients in requiring additional medical care.
This prediction was made over a considerable margin because the algorithm considered the patients' past healthcare expenditures. And black patients with the same disease as that in white patients spent less money on healthcare.
The AI prejudice was reduced to 80% with the joint efforts of researchers and Optum, a health services company.
Considering CEOs to be only male
If you Google the term CEO, you could only see pictures of males on Google. It was in 2015 that an independent research conducted by Anupam Dutta at Carnegie Mellon University in Pittsburgh revealed how Google’s online advertising system showed photos of males often for high-paying positions as compared to women.
It was suggested that the Google algorithm determined men to be more suited for high-paying positions on its own. The research also narrated that it could also result from user behavior.
How did Google respond?
Google pointed out the fact that gender is a specification that can be set while searching for this term. Even the advertisers can specify to which websites and audience a particular ad should be shown.
Effective strategies to reduce bias in AI
- Employ human-in-the-loop technology for constant improvement
Human-in-the-loop technology is based on the concept that when machines aren’t able to solve a problem, humans must step in and intervene. It helps the algorithm learn each time from the feedback by the humans and AI produces better results further.
The overall performance of the system gets improved as it learns subsequently. The dataset further becomes more accurate and safe to derive results.
- Undivided attention on data and algorithms for constant feedback
Underlying data is the major reason behind the bias in artificial intelligence models. These models can’t be trusted in the modern world if the experts don’t train them and/or revert for constant feedback to improve their decision-making capability.
- Underlying data
Data scientists should invest their efforts and attention into making diverse data that eliminates any discrepancies. It is because the data fed into the model can reflect second-order effects of historical or societal inequalities.
- User-generated data and the algorithm
Additionally, the user-generated data is also a reason behind inducing bias in AI models. These create a feedback loop when the user clicks on different versions more frequently for various searches, compelling the algorithm to display them more to the user.
It makes the algorithm also pick statistical correlations that are illegal or socially unacceptable. This information can make the algorithm put forward a biased outcome.
- Check AI decisions from a humane unbiased perspective
Making the decision of artificial intelligence models to be transparent requires some external human intervention. Traditionally, manual lead scoring models made it easy to inspect the scoring elements in the manual models that could be discriminatory in nature.
However, this practice requires more specialized skills when we are talking about AI models. Such models must be trained with the definition of fairness and how it should be computed in discussions with distinct external causes.
To counter this, the researchers guarantee AI systems of transparency by making them work on a broad range of methods like pre-processing data, integrating fairness decisions into the training process, or modifying the system's choices after the fact. A possible solution to this is "counterfactual fairness," which ensures a model's decisions are the same in a counterfactual world when delicate factors like race, gender, or sexual orientation have been changed.
- Algorithm test in a real-world setting
Algorithms are not at fault for giving biased decisions when they are trained on data that come from a specific group of people and are implemented on a different group of people.
For instance: consider your AI-powered solution is fed and trained to hire people of a certain skill only. However, you are implementing it for a group of experts with different skills. It would compel the algorithm to generate results that aren’t viable and even unbiased because it would be applying the prejudices it learned from the trained data to those for which such assumptions are not true.
In such scenarios, AI fails to give accurate results. To fix such issues in your machine learning systems, test the algorithm to identify and resolve these blunders that are only wasting your time and efforts in the long run.
The big question: can we consider AI to be unbiased ever?
It is impossible to state that no bias would exist in AI. It relies on the quality of data fed into it. In order to have this data free of any bias, it should be put into machines by an entirely impartial human mind that barely exists. Even after clearing your training data set of all the preconceptions, you may subconsciously miss out on some ideological notions with which you are not acquainted.
Since humans are the one that generates the data used by AI models, it can be said that It is unlikely to remove bias from AI in the real world.
It was reported by a 2020 State of AI and Machine Learning Report that 24% of companies stated that unbiased, diverse, global AI is critical in today’s context.
Therefore, we can take a fresh approach to reduce voice in artificial intelligence by following that AI algorithms must intervene where human bias exists to offer unbiased results.
It can be combated and brought down to a negligible level by implementing the above-mentioned steps. These are the best practices to work on the data and letting the AI derive the best yet unbiased results at the end.
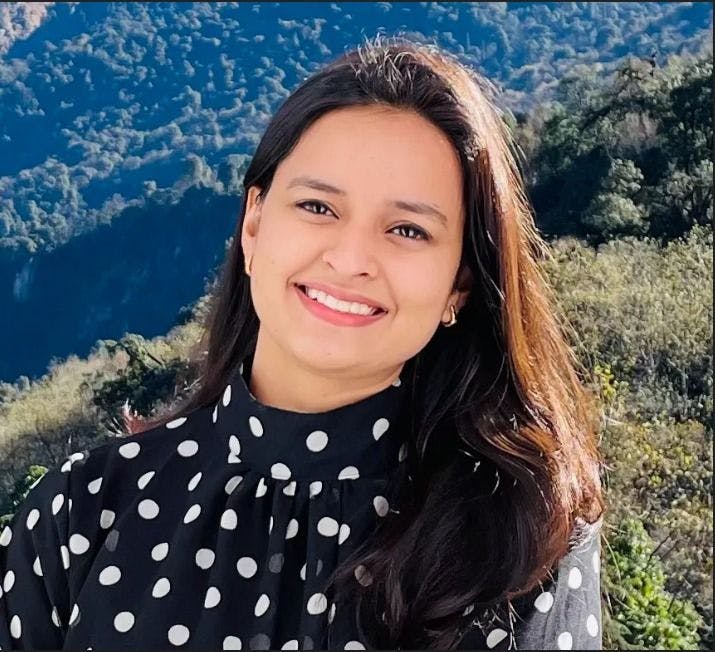
Author
Srishti Chaudhary
Srishti is a competent content writer and marketer with expertise in niches like cloud tech, big data, web development, and digital marketing. She looks forward to grow her tech knowledge and skills.