Understanding Transformer Neural Network Model in Deep Learning and NLP
•12 min read
- Languages, frameworks, tools, and trends
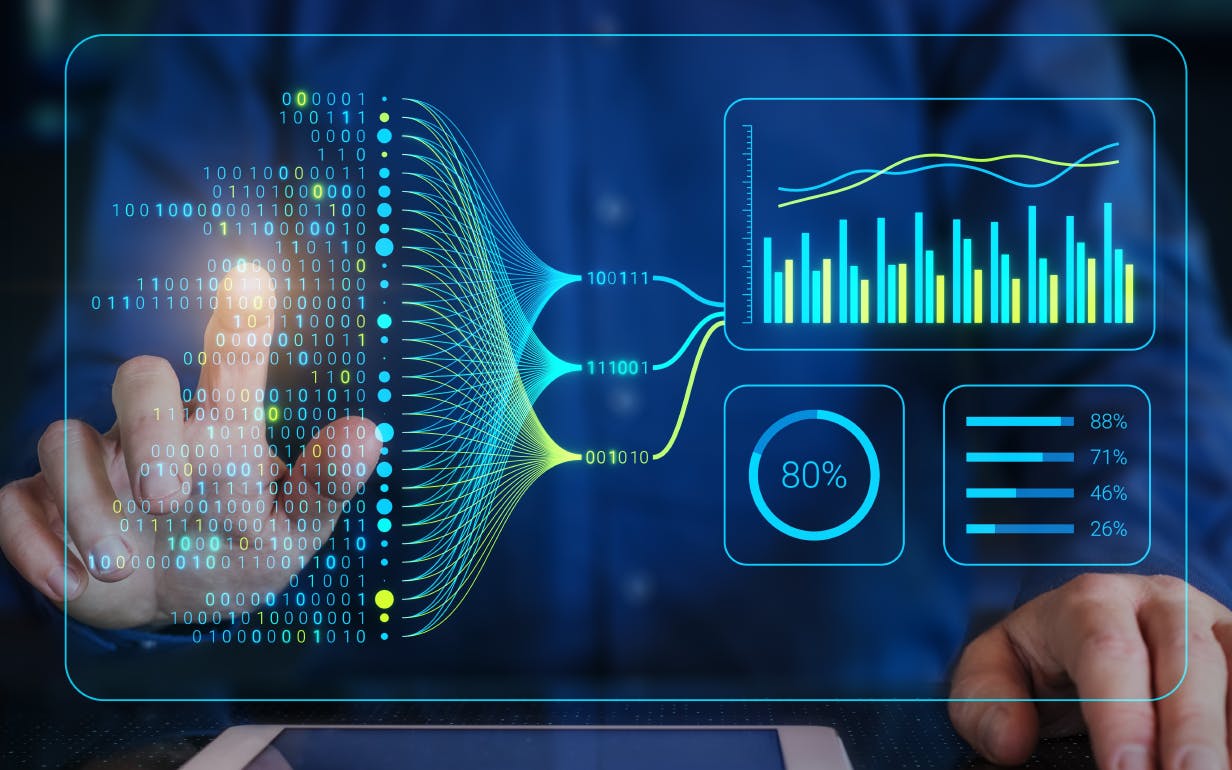
In the past few years, the Transformer model has become the buzzword in advanced deep learning and deep neural networks. This model is most suitable for NLP and helps Google to enhance its search engine results.
Since its launch in 2017, the Transformer deep learning model architecture has been evolving into almost all possible domains. This model is also helpful for time series forecasting.
Transformers are still being developed and used in various new applications by researchers. Here is a brief explanation of what makes Transformers exciting. Let's start with what the Transformer model is.
What is the Transformer model?
Transformers are neural networks that learn context and understanding through sequential data analysis. The Transformer models use a modern and evolving mathematical techniques set, generally known as attention or self-attention. This set helps identify how distant data elements influence and depend on one another.
Transformers came into action in a 2017 Google paper as one of the most advanced models ever developed. This has resulted in a wave of advances called "Transformer AI" in machine learning.
In a paper published on August 2021, researchers from Stanford identified Transformers as "foundation models" because they believe it will transform artificial intelligence.
In a recent report, researchers noted that foundation models had exceeded our expectations in size and scope over the past few years. Thus, they wrote about it as the "sheer scale and scope of foundation models over the last few years have stretched our imagination of what is possible."
Transformer model: general architecture
Transformers draw inspiration from the encoder-decoder architecture found in RNNs because of their attention mechanism. It can handle sequence-to-sequence (seq2seq) tasks while removing the sequential component.
A Transformer, unlike an RNN, does not perform data processing in sequential order, allowing for greater parallelization and faster training.
This figure illustrates the Transformer deep learning model's overall architecture. There are two main components of the Transformer:
- The encoder stacks — Nx identical encoder layers (in the original published paper, Nx = 6).
- The decoder stacks — Nx identical decoders layers (in the original published paper, Nx =6)
Models do not include recurrences or convolutions, so there is an extra layer of positional encoding between the encoder and decoder stacks to take advantage of the order of the sequence.
The Transformer encoder
An encoder consists of N layers, each of which consists of two sublayers: self-attention gets generated on the first sublayer through a multi-head mechanism.
It has been demonstrated that the multiple-head mechanism generates a final output by taking a (different) linear projection of the queries, keys, and values and producing h outputs in parallel.
In the second sublayer, we have a fully connected feed-forward network with two linear transformations interspersed with Rectified Linear Units (ReLUs) activation.
Each word in the input sequence is translated one after the other by the six layers of the Transformer encoder. However, each layer uses its own weight (W1, W2) and bias (b1, b2) parameters to function.
There is also a residual connection around each of these two sublayers. In addition to each sublayer, there is also a normalization layer, layernorm(.), whose function is to normalize the sum calculated between the sublayer input, X, as well as the sublayer output itself, sublayer(X)
Considering the Transformer deep learning architecture does not make use of recurrence, it has no inherent way to identify the relative position of words.
Positional encodings have been injected into the embeddings to inject this information. Positional encoding vectors get generated using sine and cosine functions of varying frequencies. They have the same dimension as input embeddings. Positional information is then injected by summing them with the input embeddings.
The Transformer decoder
There are several similarities between the encoder and the decoder. A decoder also has N = 6 functional layers, each composed of three sublayers:
- In the first sublayer, the decoder stack receives the previous output, provides positional information, and applies self-attention across the heads.
Decoders pay attention only to the words before them, as opposed to encoders, which pay attention to every word regardless of order. As a result, the prediction for the word at the position, i, only depends on the words preceding it in the sequence.
A multi-head attention mechanism (the implementation of multiple, single attention functions in parallel) overlays a mask over the values produced by multiplying Q and K by a scale factor.
By excluding matrix values, illegal connections are suppressed:
- Similar to the first sublayer of the encoder, the second sublayer implements a multi-head self-attention algorithm. Decoders receive queries from the previous sublayers of decoders via this multi-head mechanism in addition to the encoder's output keys and values.
- It allows the decoder to pay attention to each word in the input sequence. A fully connected feed-forward network gets implemented in the third layer, similar to the one deployed in the second sublayer of the encoder.
A normalization layer comes after the three sublayers on the decoder side. There are also residual connections between them. As described previously for the encoder, the decoder also adds positional encodings to its input embeddings.
What is the Transformer neural network?
As is well known, the Transformer plays a key role in neural network designs that process sequences of text, genomic sequences, sounds, and time series data. Natural language processing is the most common application of Transformer neural networks.
When given a sequence of vectors, a Transformer neural network encodes them and decodes them back into their original forms. The Transformer's attention mechanism is an essential component. An attention mechanism indicates the importance of encoding a given token in the context of other tokens in the input.
For instance, in a machine translation model, the attention mechanism enables the Transformer to convert 'it' into the correct gender in French or Spanish based on all relevant words. Transformers use the attention mechanism to determine how to translate words on both sides of the current word.
Note: A Transformer neural network replaces earlier recurrent neural networks (RNNs), long short-term memory (LSTMs), and gated recurrent networks (GRUs).
Transformer neural network design
A Transformer neural network takes an input sentence and encodes it into two different sequences:
- A word vector embedding sequence
- A positional encoder sequence
Word vector embeddings are numerical representations of text. A neural network, in this scenario, can only process words if they get converted to embedding representations.
Words in the dictionary get represented as vectors in the embedding representation. Positional encodings represent the word's position in the original text as a vector. The Transformer combines the word vector embeddings and positional encodings. Then it sends the combination results to various encoders followed by decoders.
RNNs and LSTMs feed the input sequentially, whereas TNN feeds the input simultaneously. Each encoder transforms its signal into another sequence of vectors known as encoding.
Decoders work in reverse order. It converts encodings back into probabilities and produces output words based on the probabilities. By using the softmax function, a natural language generates sentences from the output probabilities.
There is a component in each decoder and encoder called the attention mechanism. It allows one input word to get processed with relevant information from other words while masking the words which do not contain relevant information.
Taking advantage of the parallel computing capabilities offered by GPUs, we implement multiple attention mechanisms in parallel. A powerful GPU provides parallel computing capability. We can take advantage of this service by implementing multiple attention mechanisms parallel. This process is called the multi-head attention mechanism.
One advantage of the Transformer deep learning model over LSTMs and RNNs is that they can process multiple words simultaneously.
Feed-forward network
A feed-forward neural network is the next step. We can apply a simple feed-forward neural network to each attention vector to transform attention vectors into a form that the next encoder or decoder layer will accept it easily.
One attention vector gets accepted at a time by the feed-forward network. Unlike RNNs, these attention vectors are independent of each other, which is the best thing about them. Here, parallelization is crucial, and that makes all the difference.
Functioning in brief
Following are the functions that the Transformer deep learning model performs.
Multi-head attention
On a superficial level, imagine multi-head attention as a multi-tasker. You have a word with you, from which the Transformer deep learning model needs to learn the next word. Multi-head attention performs different parallel computations for the same word to achieve different results. These results are then connected to SoftMax to output the best suitable word.
These parallel computations might look at the tense of the word, the context of the text, the type of a word (verb or noun, etc.), and so on. The combination of these finds the highest probable word using SoftMax.
Masked multi-head attention
It is like the above-mentioned multi-head attention except that it hides the future words in the sequence relative to the word decoder is currently working on. This masking prevents the Transformer from looking into the future and realistically learning from the data.
Residual connection
The directed arrows going from one 'Add and Norm' to another without passing through the attention module is called a skip connection or residual connection. This helps in preventing the degradation of the network and helps maintain the gradient flow throughout the network for robust training.
Famous Transformers models
Most of the state-of-the-art models currently in use in the world are built using Transformers. Talking speech-to-text conversion, machine translation, text generation, paraphrasing, question answering, sentiment analysis, and so on. These are some of the best and most famous models.
BERT
Stands for Bidirectional Encoder Representations from Transformers. This technique was designed by Google for natural language processing and is based on pre-trained Transformers. Until 2020, BERT was used in almost every English-language query on Google’s search engine.
GPT-2, GPT-3
Stands for Generative Pre-trained Transformers 2 and 3, respectively. GPT is an open-source AI used for natural language processing (NLP) related tasks such as machine translation, question answering, text summarizer, and many more.
The biggest difference in both is the scale at which these are built. GPT-3 is the latest model with a lot of added functionalities to GPT-2. On top of this, it has a capacity of 175 billion machine learning parameters, while GPT-2 has a capacity of only 1.5 billion parameters.
Limitations of the Transformer
In comparison to RNN-based seq2seq models, the Transformer deep learning model made a vast improvement. However, it has some limitations as well:
- Attention can handle a particular length limit of text strings. It is necessary to split the text into segments before it can be fed into the system.
- As a result of this chunking of text, context fragmentation is caused. It is important to note that if a sentence gets split from the middle, it loses most of its context. As a result, no semantic boundary or sentence is respected in the split text.
What is attention?
Over the past few years, the attention mechanism has drawn considerable attention, especially in sequence tasks. Let's see what the attention mechanism is. According to the attention mechanism, weights are dynamically calculated based on input queries and element keys, to calculate a weighted average of (sequence) elements.
This method averages multiple elements in a simple way. However, we want to weigh each element according to its actual value, but not equally. By dynamically selecting inputs, we hope to decide which ones we will attend to more. Generally, we need to specify four parts of an attention mechanism:
- Query: In the query, we describe our search parameters or what we might pay attention to in the sequence.
- Keys: Input elements and keys are tied together, which also feature vectors. This feature vector tells us about what the element is "offering" and when it can get essentially into operation. We should design keys appropriately that will help us identify the attention-required elements based on the given query.
- Values: Besides input elements, there are value vectors as well. Our goal is to create an average of these feature vectors.
- Score function: The score function helps us to determine which elements need more attention. An input query and the key work as input to the score function, which outputs the query-key pair's score/attention weight.
The most common implementation is a dot product or a small MLP (Multi-layer Perceptron) that compares similarity metrics.
We use the softmax function on all score functions output to calculate the average weights. Therefore, we give higher weights to the value vectors having a key closest to the query. By using pseudo-math, we can express it as follows:
What are sequence models? A general introduction
Our complete life is a course of events that either we or our circumstances control. You can spot countless patterns anywhere you look. Something that occurs with the dependency on its predecessor is termed a sequence. For example, in a sentence of any language, all the words have a predefined structure to deliver a message. These sentences are nothing but a sequence of different words.
In deep learning, we encounter sequences very frequently in a dataset. The factor that concludes whether to use a sequence model or not is the presence of other highly correlated features in the dataset and what we are expecting from the model as an outcome.
We use sequence models to learn the sequence order and how those elements interact with one another throughout the period. This helps to forecast the sequence in the future. The most popular design for such models, before the invention of Transformers, were Recurrent Neural networks (RNNs), Long Short Term Memory Networks (LSTM Networks), and Gated Recurrent Units (GRUs).
Irrespective of their exceptional performance and well-adopted application, these models had bottlenecks. These were unable to learn long-distance dependencies within the sequences to maintain an appropriate context. With such issues and increasing demand for quick solutions, scientists came up with a concept called “Attention”. In Transformers, we work very closely with self-attention. To read in detail about these concepts, check out the paper: Attention Is All You Need.
Reinforcement learning using Transformers
Reinforcement learning is a goal-oriented algorithm that rewards every correct step towards achieving a complex goal. The incentivization method reinforces the right decisions. Long Short-Term Memory (LSTM) is an effective and mostly used technique for Transformer reinforcement learning.
On the other hand, Transformers are effective for NLP tasks (consider GPT and BERT). Transformers in NLP try to solve sequence-to-sequence tasks by handling long-range dependencies. To handle reinforcement learning tasks, Transformers are the most preferred choice.
The Markov method of reinforcement learning is the most preferred technique for on-time task completion. Although to predict what action sequence will result in a high reward sequence, we can use a sequence modeling problem.
Models with high capacity and power that work well with other domains, like NLP, can provide better Transformer reinforcement learning solutions.
Recently, University of California, Berkeley researchers reported how we can use state-of-the-art Transformer architectures to simplify reinforcement learning by making it a ‘one big sequence modeling’. It is based on distributions of rewards and actions over sequences of states.
In their paper, “Reinforcement Learning as One Big Sequence Modeling Problem,” they simplified the design decision-making process, for example, by removing the need for separate behavioral policy constraints.
As a result, we can apply this approach to various domains, including dynamics prediction, long-horizon dynamics prediction, and offline reinforcement learning.
Conclusion
To conclude this article, we saw what sequential models are, how Transformer deep learning models were developed later to all the other types of sequential architectures, and how and why they proved themselves to be the most powerful among all.
Then we delve deep into the Transformer’s composition and their work behind the scene, followed by some of their most popular applications. Moreover, you can find even more examples where you can see how useful and reliable they are.
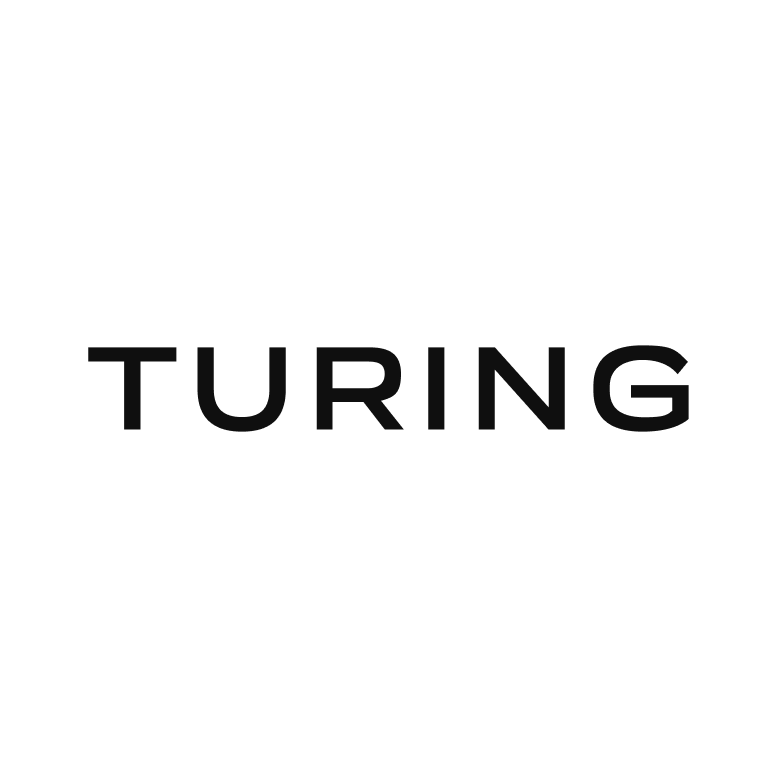
Author
Turing Staff